Use of real-world evidence (RWE) in support of regulatory filings continues to be a key focus area among life sciences companies. As the field learns from regulator feedback about how RWE can meaningfully contribute to submissions, we begin to get a clearer picture of near-term opportunities for regulatory RWE. This session included presentations from life science leaders about how their organizations have successfully applied RWE in recent regulatory submissions, as well as a presentation on Flatiron’s evolving perspective on the use of RWE for regulatory use.
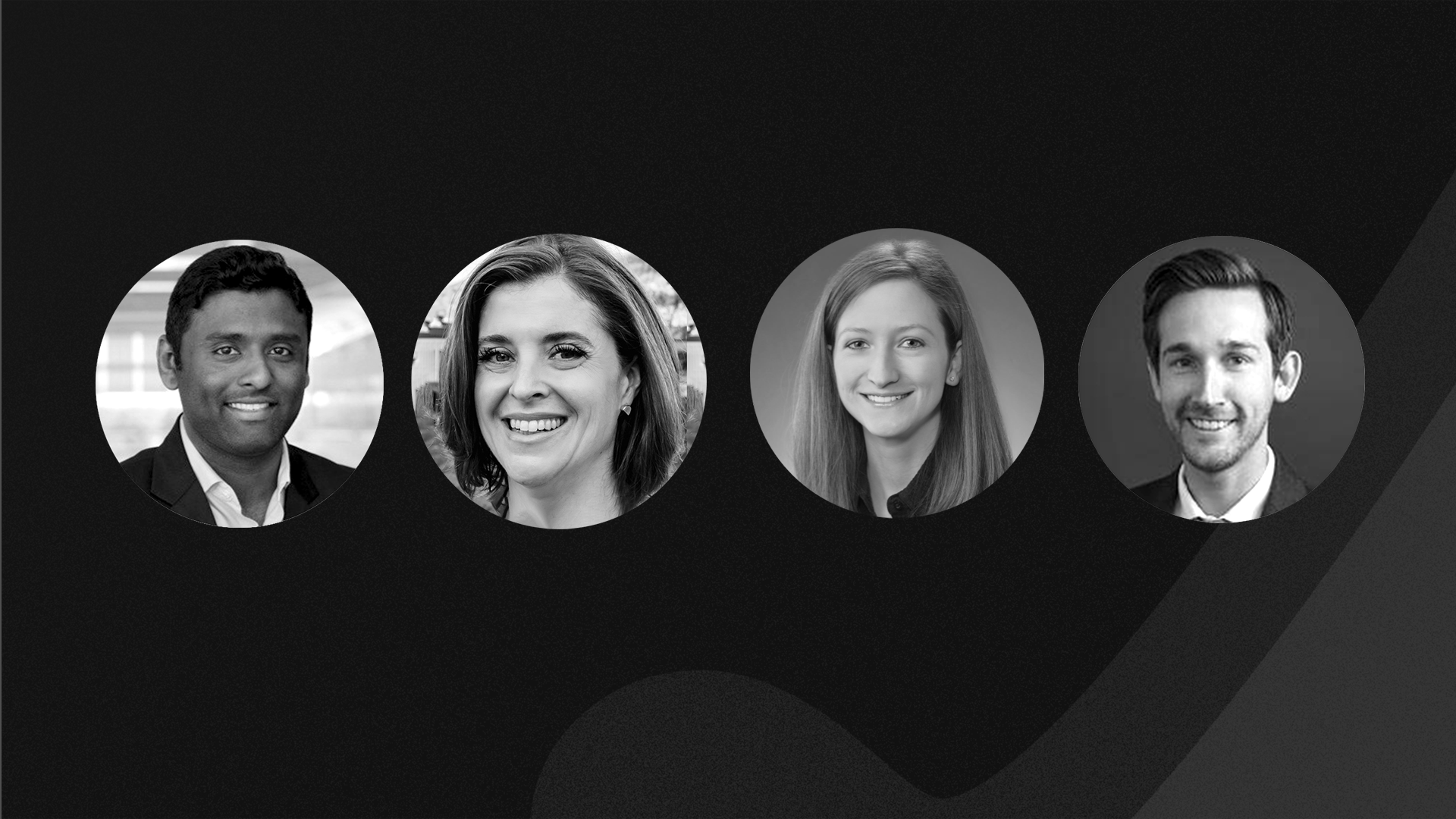
Transcript
Christopher Gayer: Thank you so much, everyone in attendance here, for joining us today to be part of this exciting conversation on near-term opportunities for regulatory real-world evidence. My name is Chris Gayer. I'm a Director of Product Management focused on regulatory real-world data here at Flatiron Health. I'm really excited about this webinar today, and even more excited to introduce you to our speakers. So today's session is going to include Dr. Pranav Abraham, Director of Global Health Economics and Rare Hematology at Bristol Myers Squibb, Dr. Lynn Howie, Medical Director here at Flatiron Health. Dr. Kristin Sheffield, Research Advisor at Eli Lilly and Company, and you'll get to hear from each of them shortly. But first, a few quick housekeeping items before we dive in.
I'd like to draw your attention to the Q&A option available throughout today's webinar. If you hover over your screen with your mouse, you'll see an option for Q&A towards the bottom middle of your screen within the black bar. At any point during today's presentation, feel free to submit a question through this feature. While we do have a packed agenda, we have made sure to save time toward the end of our session for you to ask your questions to the speakers using this function. Please feel free to reach out to us after the webinar with any follow up questions or discussion you may like pertaining to the details of today's conversation. And also, if you have any technical issues or questions throughout this seminar, please let us know via the Q&A tool and we'll do our best to assist you. And before we jump in, please do excuse any interruptions from our pets or our loved ones as many of us are still working from home.
We have a really great agenda lined up today. First, Lynn is going to kick us off by setting context for today's discussion and by providing an overview of the near-term opportunity areas for leveraging real-world evidence in the regulatory space. Pranav will then present a use case where BMS successfully leveraged real-world data, providing natural history information and regulatory context, followed by Kristin, who will present a case on Lilly's successful use of real-world data to fill evidence gaps related to dosing in the post-approval space.
Before we get started though, we'd love to learn a little bit more about you, our audience, with a poll. So you should see a poll pop up on your screen momentarily. If you have two monitors, please be sure to check both as the poll might appear on your other screen. Other attendees will not be able to see the responses that you choose. So our first poll question today is, which of these applications of real-world evidence has your organization historically considered or incorporated into regulatory submissions? Please select all that apply. Characterizing natural history or unmet medical need, as an external comparator to a single arm trial, to expand a label into a new indication, to satisfy a post-marketing requirement or commitment, or none of the above. We'll give this about 10 more seconds and then share some results.
All right, let's end the poll and see what we have. Very interesting. Okay. So not surprising to see most endorsement for natural history, unmet medical need, and as an external comparator, but really a close second for uses of label expansion into new indications and satisfaction of postmarking requirements or commitments. So I think this poll is a really nice transition then into our featured presentations today. And with that, I am very excited to introduce Lynn, who's going to present near-term opportunities to leverage real-world evidence for regulatory use. Lynn, take it away.
Lynn Howie: Thanks so much, Chris. Before turning it over to our industry partners to discuss their recent experiences using real-world data to support regulatory decisions, I'd like to start by setting the stage. As this audience knows well, our understanding of the diagnosis and treatment has changed rapidly in the last century. We have evolved from understanding tumors based on their anatomical site and tissue of origin and treating them primarily with systemic cytotoxic therapy, to molecular characterizations of tumors where targeted therapies, such as monoclonal antibodies, tyrosine kinase inhibitors, and immunotherapies can be used to personalize treatments based on a tumor's characteristics.
As our treatments get more nuanced, so too does our need for real-world evidence to understand patient outcomes and experience. These data are critical to understanding the natural history of disease, to provide comparisons for increasingly small subsets of patients with rare disease types, and to help drive drug development and innovation in a space of rapidly evolving therapies. real-world data can serve to complement traditional randomized controlled trials and expedite the availability of therapies advancing the fundamental goal of oncology, to provide patients the right therapy at the right time, to maximize tumor control and extend life with minimal toxicity.
There has been significant momentum and learning at the intersection of real-world evidence and regulatory decision making. Following the mandate outlined in the 21st Century Cures Act of 2016, FDA released a draft framework for real-world evidence and subsequent guidances around the use of this evidence in regulatory submissions and scenarios where these data may provide support for regulatory decisions. Since the introduction of that framework, we've seen numerous examples of successful and unsuccessful uses of real-world evidence in regulatory submissions. More importantly, we've had the opportunity to learn from those reviews and to specifically understand how regulators evaluate the use of these data in different clinical and regulatory contexts. Understanding how to use real-world evidence and regulatory submissions is growing further as a result of the recent release of a set of new FDA draft guidances. Since September, we've had two new draft guidances, the first focusing on EHR and claims data use for supporting regulatory decision making, and the second on data standards. We're expecting two more real-world data specific guidances by the end of the year that will further shape our collective understanding of what regulatory ready real-world evidence looks like to FDA.
Speaking now to Flatiron's experience over the past few years, our real-world data have been used to support over 12 briefing packages, 7 information requests, and have been included in at least 14 submissions. Flatiron Health has joined our life science partners in 7 health authority meetings as well. During the same time, we've received regulator feedback from FDA, EMA, and PMDA on the utility of Flatiron real-world data in 22 unique submission opportunities with more than a dozen partners. These direct engagements and feedback from regulators have provided a source for many learnings about where real-world data are and are not most helpful for therapeutics developers in the current state.
Regulators, including FDA, have indicated that use cases where real-world data are most likely to have impact include those diseases where there's a significant unmet need and there are few available therapies. Rare patient populations where randomized trials are not feasible, use cases where there is an expected large effect size based on preliminary data, and use cases to fill in evidence gaps where there is already a substantial body of evidence surrounding the safety and efficacy of a therapy in a related patient population.
While the holy grail of real-world evidence may be to provide an external or synthetic control arm, which I'll refer to as a formal comparator, for a small single arm study to potentially lead to a regular approval with substantial evidence of efficacy, there have been multiple valid issues raised by regulators that limit the near-term viability of leveraging electronic health records-based retrospective real-world evidence for this use. The feedback from regulators has highlighted common challenges with real-world data that are critical to consider for your use cases. These include endpoint definition and measurement. In use cases involving a direct statistical comparison between a real-world cohort and a clinical trial population, the lack of standardized approaches to objective assessment of outcomes in the real world, as well as the lack of understanding of the concordance between real-world and trial-based measures, limits the interpretability of real world and trial cohort comparisons, as well as the overall confidence and study results generated from real-world endpoints. Real-world data missingness. As retrospective data are not being captured as they would be in a trial, the availability of certain data elements, for example, ECOG score at index, may be limited, making the comparison of real-world to trial cohorts challenging and potentially limiting our confidence in conclusions.
Cohort comparability. Variables that are not captured routinely and consistently in clinical care cannot be used to inform cohort selection or quantified to understand their impact on outcomes, which undermines our ability to make causal inferences. Additionally, attempts to maximize cohort comparability can lead to small cohorts, which themselves lead to underpowered analyses and unstable and uninterpretable estimates.
At Flatiron, we're doing all that we can to improve our underlying real-world data product to address these concerns where we are able. Additionally, it is critical that the collective “we”, meaning data providers and regulators, continue to work together, along with our colleagues at Friends of Cancer Research, Duke Margolis and the Real-World Evidence Alliance, to move the field forward.
Despite this, there are multiple use cases where we've seen that real-world data can be used in support of regulatory applications right now. Across the drug development cycle from clinical development through post-approval, we have seen real-world evidence successfully used to support regulatory decision-making. Based on our regulatory feedback to date, it's very clear that the likelihood of acceptance of real-world evidence varies by how this evidence is used to support a regulatory filing.
This perspective is informed by our evaluation of existing precedent, FDA guidances, as well as our own experiences engaging with partners and regulators around specific real-world evidence opportunities at the submission and project level.
So where is real-world evidence adding value? Generally speaking, we've seen customers realize the value of real-world evidence in the clinical development phase frequently, using these data to inform comparator arm selection for clinical studies and inform cohort definitions. We've additionally seen a number of instances where our partners have succeeded in developing real-world evidence benchmarks. We define this as the use of real-world data studies for contextualization and characterization of natural history of disease among similar populations at the aggregate level. This can help describe evolving treatment patterns and outcomes, and we believe that this is an opportunity area for near term real-world evidence acceptance, and we'll hear more about a successful use case from Pranav using data this way.
In the post-approval phase, we've now seen several exciting applications of real-world evidence as supportive evidence to fill gaps in understanding the safety or effectiveness of approved products. Examples of opportunities in this space include the potential label expansion of a therapy into a new indication for a rare or unique population, the use of these data to help better understand the contribution of an approved agent and a novel combination, and also potential label changes that can help inform dose, dosing regimen or route of administration, an example of which we'll hear more about in Kristin's upcoming talk. We believe that the use of real-world evidence in the post-approval space is a major opportunity area for near-term acceptance. So despite limited traction with the formal comparator use case in oncology approvals to date, there remain key opportunities for real-world data to help fill important evidence gaps needed by regulators across a variety of use cases. And with that, I'm delighted to turn the mic back over to Chris to introduce our guest speakers.
Christopher Gayer: Awesome. Lynn, thank you so much. I think that really set the stage nicely for our next couple of speakers. And now, I'm really excited to turn things over to Pranav from Bristol Myers Squibb to share how real-world data can be used to provide information on natural history and disease. Pranav, it's all yours.
Pranav Abraham: Perfect. Thank you, Chris. Can you hear me well? Perfect. Welcome, everyone. I'm Pranav Abraham, part of the Worldwide Health Economics and Outcomes Research Hematology Team here at Bristol Myers Squibb. Though I'm part of the Hematology Team now, over the past several years, I've had the opportunity to lead multiple solid tumor indications, and the work that I'll be presenting today has been a part of my previous role. So before I start, today, I have the privilege to present a case study on how we were able to generate real-world data as natural history information and strengthen our regulatory filing in the US for second line esophageal squamous cell cancer. I would like to acknowledge that the strategy, execution, and outcome of this filing was due to a larger team effort. And though I have the opportunity to present this today, I would like to recognize all my colleagues that were involved in this work stream. Next slide. Thank you. So before I jump right in, just to provide everybody some context. First, why was there a need for real-world data during a regulatory filing for esophageal squamous cell cancer? Let me walk you through some of the key aspects of this clinical trial design, which is ATTRACTION-3. So ATTRACTION-3 was a phase three randomized study of nivolumab versus docetaxel monotherapy in patients with esophageal squamous cell cancer, who were either refractory or intolerant to fluoropyrimidine and platinum-based combination chemotherapy. All the 419 patients in the clinical trial had a performance status of 0 or 1, were randomized into two treatment arms as you see.
210 patients received nivo while 209 received taxane monotherapy. All patients in this study continued treatment until progression, all conditions that were unacceptable in view of their safety. The primary objective of this trial was to assess overall survival and compare it among treatment arms. I won't go over all the other endpoints. They have been listed right below. So this trial, when it read out, showed that patients treated with nivo monotherapy had superior overall survival compared to those patients that received taxane monotherapy, which is either docetaxel or paclitaxel. Now, the challenge here was that this trial only had 18 Western patients out of a total of 419. Although the trial was designed and conducted as a global trial, delays in site activations, coupled with low prevalence of the disease in participating Western countries resulted in a very limited number of patients enrolled outside of Asia. So we as a team within BMS anticipated questions from regulatory bodies around the applicability of ATTRACTION-3 to Western countries, and hence, our objective was to showcase the relevance of ATTRACTION-3 clinical trial results to medical practice here in the U.S., by supplementing it with U.S. real-world data. Next slide. So before we started analyzing any of these multiple available real-world datasets, we wanted to first identify some key evidence gaps, and we identified that very few articles reported real-world outcomes for esophageal squamous cell cancer. And I particularly remember back during that time in 2019, there was no study that reported U.S. real-world outcomes for esophageal squamous patients. And this could mainly be because the prognosis for this disease was found to be very poor, and the fact that esophageal adenocarcinoma is more prevalent than squamous cell carcinoma here in the U.S., and likely in Western countries.
At that point in time, I also remember there was no standard of care in second line. Real-world outcomes for these patients were uninvestigated, and there was a need to characterize the treatment patterns and compare outcomes for patients treated in line with NCCN guidelines with those from ATTRACTION-3. Hence, our key research questions focused on better understanding the clinical characteristics, treatment patterns, survival outcomes among all patients who received two or more lines of therapy. This is among esophageal squamous cell cancer patients. It was also critical to compare overall survival of advanced or metastatic patients who received second-line taxane therapy with those who received non-taxane second-line therapy, and this was particularly done because chemotherapy patient cohorts in ATTRACTION-3 were randomized to only receive taxane monotherapy or nivo monotherapy. Next slide.
So using the Flatiron dataset, we conducted a retrospective analysis. First, we identified all patients being treated in the U.S. for advanced metastatic disease, specifically esophageal squamous cell cancer. I remember we identified close to 300, 350 patients. I see that the number is not here, but again, since second line is the focus, we found 86 esophageal squamous cell cancer patients treated with second-line therapies between 2011 and 2019. And these 86 patients matched our inclusion-exclusion criteria, which the main ones have been listed below. I won't be going over them one by one in interest of time, but I would say that to the extent possible, we ensured that this cohort resembled patients in ATTRACTION-3. We then further classified patients that received second-line therapy into two buckets, patients that received taxane-based treatment. This was specifically done in line with the then NCCN guidelines.
Just to provide more perspective, if I may take your attention to the table on the right, the key characteristics and outcomes from both our ATTRACTION-3 trial and Flatiron data analysis, you will find that the median age, the proportion of males across the taxane arms in ATTRACTION-3, and the Flatiron data analysis were found to be very comparable. We also found that the majority of patients in the Flatiron dataset were Caucasians. This meant that the characteristic makeup of patients with advanced or metastatic squamous cell carcinoma in the East and the U.S. can be very similar. When I move on to outcomes, when you look at the median survival from initiation of second-line treatment, it was found to be 6.7 months for all second-line patients, whereas it was comparable, which is 8.4 months among patients receiving taxane therapy in ATTRACTION-3, and 7.3 months among those receiving taxane therapies in the Flatiron dataset.
Even when we compare the landmark survival, let's say assessed at the 12-month mark, a similar proportion of patients that is 34% in the taxane arm in ATTRACTION-3 were alive compared to 24% in the taxane cohort in the real-world Flatiron dataset. Now, when these proportions were compared to the proportion of nivo patients alive at month 12, the benefit of nivo monotherapy was quite evident and was found to be superior. So these results in a whole gave us confidence that survival, first of all, could be comparable across regions, at least in the advanced metastatic stage esophageal squamous cell carcinoma. They also highlighted those patients in the ATTRACTION-3 trial that received nivo had superior outcomes, and these clinical trials could be applicable to U.S. medical practice. Next slide.
So what did we really learn from carrying out these analyses and how did it really make an impact for patients? Let me highlight some key insights. First, only a quarter to a third of patients treated in frontline after diagnosis of advanced or metastatic disease for esophageal squamous cell cancer went on to get second line treatment. So that's a very small proportion. Now, with such a small proportion moving on to later lines of treatment, coupled with poor survival outcomes of existing treatment options, this showed that outcomes of palliative chemotherapy for advanced disease were only modest and offered very poor, long-term survival. This helped us to highlight an urgent unmet need for new treatment options in the setting, which is the second line. I personally feel that real-world evidence can certainly strengthen, provide more perspective to clinical trial results, which can aid in better clinical decision making.
In our case, it showed that demographics and clinical characteristics of ESCC patients with advanced disease were comparable across Asia and the U.S. So regardless of region or ethnicity, advanced ESCC was an aggressive disease and is associated with poor prognosis. Now, to move on to the impact we were able to have with these real-world data analyses, these Flatiron analyses, along with other real-world analyses that we had conducted, strengthened our U.S. regulatory filing, which ultimately led to nivo being approved in June 2020. This was the first and I believe still remains the only IO therapy approved in the U.S. for second-line esophageal squamous cell cancer, regardless of PD-L1 expression. So that sums up my presentation and how we were successfully able to use real-world data in our interactions with regulatory bodies and in our submission package. I would now pass it on to Chris to introduce the next presenter.
Christopher Gayer: Excellent. Thank you, Pranav. That was a really insightful presentation and a really innovative use of real-world evidence to strengthen your filing. Okay. So for our final presentation, I'm really delighted to turn things over to Kristin from Eli Lilly to educate us on how they leveraged real-world data to fill evidence gaps related to dosing in the post-approval space. So Kristin, take it away.
Kristin Sheffield: Thank you. So good afternoon. My name is Kristin Sheffield, and I'm a Research Advisor within our Global Patient Outcomes and Real-World Evidence Organization at Eli Lilly, and today, it's my privilege to present an example illustrating how real-world data may be used to fill evidence gaps in the post-approval space. And I would like to recognize and acknowledge the team of colleagues at Lilly who led this effort. Before I begin, I just want to make clear that the views and opinions expressed in his presentation are my own. Next slide, please. So Erbitux received initial approval from the FDA in 2004 for the use in the treatment of metastatic colorectal cancer with a 250 milligram per meter squared weekly dose. Biweekly dosing cetuximab at 500 milligrams has been shown to closely mirror the exposure of the 250 milligram weekly schedule, based on pharmacokinetic exposure data from a phase one dose escalation study. The biweekly dosing schedule is recommended by current international guidelines, such as the NCCN, and it's commonly used in clinical practice.
So there are several potential benefits of a biweekly dosing schedule. In clinical practice, biweekly administration could reduce the burden for patients and medical staff by allowing infusions to be scheduled with other biweekly chemotherapy regimens, potentially reducing the number of patient visits, and the biweekly dosing schedule may lead to a reduction in drug wastage and costs as well. Next slide, please. So I'll briefly provide some background information about the Model-Informed Drug Development Program, because this is the mechanism used by the team to pursue the label change. MIDD is a pilot program that allows drug developers to discuss with FDA the application of exposure-based biological and statistical models derived from preclinical and clinical data to the development and regulatory evaluation of medical products.
And MIDD approaches can optimize drug dosing in the absence of dedicated trials. So the team applied and was accepted in the pilot program and granted two meetings with the FDA. And at the bottom of the slide are some additional examples of how the MIDD pilot program has been used to change dosing regimens and reduce the infusion time for other drugs. Next slide. So the primary evidence in the submission was the population pharmacokinetic modeling and simulation analysis that compared predicted exposures of cetuximab 500 milligrams biweekly to observed cetuximab exposures in patients who received 250 milligrams weekly dose. However, these analyses lacked the treatment exposure response data from cetuximab trials. So to supplement these results, additional supportive evidence was included in the submission, and that included a systematic literature review and meta analysis, which was conducted for clinical studies of patients who received weekly or biweekly cetuximab.
Lilly also submitted an observational cohort study comparing overall survival between weekly and biweekly dosing schedules in patients with metastatic colorectal cancer treated with cetuximab. That's what I'll discuss today. Next slide. Thank you.
The study included patients with stage four or recurrent metastatic colorectal cancer diagnosed on or after January 2013. Patients received first-line, second-line, or third-line treatment with cetuximab plus or minus FOLFIRI, FOLFOX, or irinotecan. Patients had documentation of KRAS wild type status and must have initiated treatment at least six months prior to the end of the database at the time of study conduct.
Patients were assigned to weekly or biweekly cohorts in a line of therapy if they had 70% or more cetuximab infusions with a gap of four to 10 days, or 11 to 18 days respectively from the previous infusion in that line.
One-to-one propensity score matching was used to balance the two cohorts according to baseline clinical and demographic variables. Propensity scores were generated for the line-agnostic overall population, as well as separately for each line of therapy.
Patients were followed from the initiation date of the cetuximab-containing regimen until the end of activity, death, or the end of the database. The primary endpoint was overall survival, and the secondary endpoint was time to treatment discontinuation. In the interest of time today, I'll focus on the mortality results. Next slide. This table shows that there were 1,075 patients in the overall study sample. Approximately 60% of patients received the weekly dosing schedule with a median dose that was close to 250 milligrams per meter squared. Around 40% of patients received a biweekly dosing schedule with a median dose around 485 milligrams per meter squared. These estimates were similar across the first, second, and third lines of therapy. Next slide. After the propensity score matching, the weekly and biweekly cohorts were well-balanced, and Kaplan-Meier overall survival curves are presented here comparing the dosing schedules for the overall population as well as by line of therapy. The blue line represents the biweekly dosing, and the red line is the weekly dosing.
Hazard ratios were generated using Cox proportional hazards regression models, and they represent biweekly compared to weekly dosing. So no significant differences were observed in overall survival in the overall population or by line of therapy. Next slide.
A number of sensitivity analyses were conducted in anticipation of questions from the FDA during the initial meeting, as well as to address FDA comments that were made during that meeting. This table shows just a selection of the sensitivity analyses for the overall population that were conducted.
For example, more stringent rules were applied for classifying patients into dosing cohorts, so requiring that 100% of infusions fell within the respective time intervals for weekly or biweekly dosing.
Another sensitivity analysis excluded patients with large gaps between infusions. There was another analysis that excluded patients with missing performance status, and that was around 38% of weekly patients and 29% of biweekly patients. 1:2 matching ratio of biweekly to weekly patients was also explored. Then another sensitivity analysis involved the use of entropy balancing rather than propensity scores to balance the two cohorts.
I'll note here that the hazard ratio was significant for the overall population, favoring the biweekly cohort. But the hazard ratios for each line of therapy were not statistically significant.
Not shown here, an analysis that compared outcomes between dosing cohorts within each cetuximab-based treatment regimen. For example, among patients who received cetuximab plus FOLFIRI. Next slide.
The study had several limitations that we should note. As many of you know, propensity score methods only address measured confounding. There's the potential for residual unmeasured differences between patients that could influence the study results if they're associated with both choice of the dosing schedule as well as the outcomes. Related to this point, data availability was limited to what was documented in the database, and some important variables like performance status were incomplete. The analyses also did not account for time-varying confounders, such as changes in treatment patterns over time.
Then finally, due to the line of therapy rules around cetuximab, patients were permitted to enter the study cohorts up to 60 days after the start date of the treatment regimen. So technically, the time from the start of the regimen to the start of cetuximab, if these are different dates, is considered immortal time, in which patients could not have had an event. Next slide please.
In conclusion, there were no significant differences observed in overall survival associated with biweekly and weekly dosing schedules in the main analyses for the overall population and by line of therapy.
The findings were robust to a number of sensitivity analyses. The one exception that I noted previously is when entropy balancing was used to control for bias. The hazard ratio was significant, favoring the biweekly dosing schedule.
FDA emphasized that the pharmacokinetic modeling analyses were the primary evidence, and the real-world evidence results and meta-analysis of clinical studies were supportive in the overall assessment of dosing schedules. This is consistent with the nature and the purpose of the MIDD pilot program.
Then finally, FDA reviewers demonstrated a strong understanding of the real-world data and provided insightful comments on the analyses, including suggesting sensitivity analyses and thoughtfully considering potential limitations and how they could impact study conclusions.
Now I'll turn the time back to Chris.
Christopher Gayer:
Excellent. Thanks so much, Kristin. Great presentation and really interesting use case.
We're going to do one more poll question before we move to Q&A. We'd love to get a sense from you, the audience, after hearing from today's speakers, how folks are feeling about future applications of regulatory real-world evidence. Our last poll question here is more future-focused. Let's say over the next two or so years, we'd love to know which regulatory applications of real-world evidence you think either you personally will be thinking about or believe that your organization should be considering the pursuit of. Again, please select all that apply: characterizing natural history or unmet medical need as an external comparator to a single arm trial, to expand a label into a new indication, or to satisfy post-marketing requirements or commitments, or none of the above. We'll give it about 10 seconds and take a look at the results of the poll.
Okay, let's end the poll and share the results. Very cool. I do see some movement in the data from before the discussion toward afterwards. Still a high level of conviction and interest in real-world evidence to support natural history studies and as external comparators in support of single arm studies. And absolutely some interest and new interest, it would seem, in the expansion of labels and the new indications and in the post-marketing space. Really interesting data. Thanks to the audience for sharing that with us.
I think we can now transition over to the Q&A segment of today's session. I would love to kick things off with a question for you, Kristin. I wonder if you can let us under the hood a little bit on the strategy over at Eli Lilly, as you are thinking about this. More pointedly, how did your team decide to use real-world data for this application?
Kristin Sheffield: Sure. Once the decision was made to move forward with an application to the Model-Informed Drug Development Pilot Program, the team considered the different options in terms of types of evidence and potential data sources. I think the team recognized that the PK models would be the primary evidence, but they knew there were some limitations. They had some experience using electronic health records and claims data to describe the use and outcomes of cetuximab. So they believed it would be feasible to evaluate dosing schedules using real-world data and that the real-world evidence would provide useful, supportive information to strengthen the overall submission package.
Christopher Gayer: Got it. Thanks for sharing. Next question for you, Pranav. Can you share either advice or insight on how you and your team thought about defining the cohort criteria for the real-world data study cohort? We spend a lot of time thinking about the appropriate cohort definition, whether that cohort's in support of natural history or even in the context of an external formal comparator. How did you and your team think about cohort criteria and what the right level of specificity was for this use case?
Pranav Abraham: Right. Chris, so that's a great question. When we started looking at different data sets, I mean, I believe we looked at a couple of things. First is to make sure that the data that we wanted to use is diverse enough so that you know it's applicable to the geographical location that we wanted to submit to. The setting in which the real-world data is collected is representative of how a majority of patients are treated in that geography location. Third was really trying to seek clinical input, if necessary, so as to understand how patients are treated in the real world.
We were able to develop algorithms that could accurately identify different lines of treatment. This is not just limited to systemic treatment, but also, let's say, radiotherapy and maybe surgical treatment if they are part of how patients are treated, depending on where they are in the disease. It may be early stage disease or advanced or metastatic.
Lastly, in my experience with real-world data, I believe we as a team, we had to be realistic and transparent. What I really meant by that is being aware of the limitations and, to the extent possible, understand the nuances of how certain diseases or cancers are diagnosed or staging of that disease is carried out.
Finally, how all of this data is really captured and where it is captured, whether structured, unstructured, nuances like these. Because of all of these nuances, and when we look at real-world data, this could eventually deviate from clinical guidelines.
Those are some of the things that we had to keep in mind while finalizing the dataset or what data we should really use for our submission.
Christopher Gayer: Thanks for that, Pranav. Clearly a lot of thought went into the thinking and decision making around cohort definition. I also really appreciate your point around the importance of transparency and clarity around any limitations in your ability to define a cohort, something we think about a lot and often advocate for, particularly when it comes to conversations with the agency about how the cohort is being defined and what the strengths and limitations of that approach are. Really spot on. Thanks, Pranav.
I think that's a nice transition to a question for you, Lynn, which is can Flatiron offer some really more procedural recommendations on how life science companies can position themselves for success when submitting a regulatory application containing real-world evidence?
Lynn Howie: I think what we've learned time and time again is that to successfully use these data for evidence, you have to treat them in many ways the same way that you would a clinical trial. That means meeting with FDA to discuss your plan and pre-specifying your plan with a protocol and a statistical analysis plan. Not that dissimilar from how you would a typical clinical trial.
Getting alignment early from FDA and discussing all of the issues surrounding what is going to be your outcome assessment, what additional measures are needed in an effort to help bolster confidence in your data are all critical.
So I encourage people, when they think about use of real-world data in their evidence packages, to discuss this early on with FDA, especially if there is going to be a substantial kind of claim based on the real-world data so that there can be early alignment and discussion of the potential pitfalls upfront as well as clarity on the part of regulatory authorities that the data are not kind of subject to a look or other things that might raise regulatory concerns about being able to rely on what the outcomes are.
Christopher Gayer: Excellent. That's nicely said. Pranav or Kristin, anything you'd want to add in response to that question based on your experiences?
Pranav Abraham: I think for me, I specifically remember when we started putting this together and trying to create analyses and have these evidences ready. One point to what Lynn just mentioned and when I go through the guidance document that was recently published, right? So, it's actually good to see that the FDA has laid out guidances specifically on data capture, missing data, data linkages, unstructured data, definition of certain outcomes, co-variants, how do you, in terms of treatment effect modifiers, what do you do? So, all of this guidance is really good to see because I feel that there would be more and more use of such real-world use cases of real-world evidence. Specifically for us as a team here at BMS, we engaged with the FDA, remember, very early. This data was presented as part of our Pre-BLA Type A Meeting or in simpler terms, part of the pre-submission package we presented to the FDA. The statistical analysis plan, the protocol, and early results from these studies. And then, it was encouraged to be included in the final submission packet. So yeah, that would be my two cents.
Christopher Gayer: Yeah. That makes a lot of sense. Thanks. Thanks, Pranav. All right, I'd love to pivot to another question for you, Kristin. How would you describe your interactions with the agency, you and your team's interactions, of course, specifically in relation to this supportive real-world data study that you presented on? I believe in your talking points, you mentioned some insightful comments or sort of insightful engagement with the agency as you pre-wired and tested what would be acceptable from their perspective. So, did anything surprise you or what can you share with us about that experience engaging with, related to this application?
Kristin Sheffield: Sure. So, the MIDD Pilot Program involves a paired set of meetings with the FDA to discuss the proposed submission package. And as I mentioned, FDA provided very useful comments and they asked for several sensitivity analyses related to the dosing schedules themselves and how patients are classified into dosing schedule. Patients who didn't fit into the original dosing scheduled definition. They also asked questions about potential unmeasured confounding. And like I said, the reviewers also demonstrated in depth understanding of the Flatiron Health database and the rules used to derive lines of therapy. So, for example, FDA commented on how the line of therapy rules allow cetuximab to be added within the first two months after the start of the regimen and asked Lilly to consider potential bias that could result. And they also conducted their own analyses and used the SAAS files and patient-level datasets that we provided to them.
Christopher Gayer: Really interesting to hear just how dug in the agency was to the real-world data in this context. Pranav, I wonder if you have any similar insights to share or any surprises on your side as you engaged with the agency.
Pranav Abraham: I think for us, Chris, as I mentioned during my presentation, this was just one part of the entire data package. So, we did multiple real-world data analyses because if you remember the numbers that I showed, right? So, esophageal squamous is just the smaller part of the whole esophageal cancer. At least here in the US, the prevalence is really low. So, we've had to do multiple data analyses where it's just not limited to patients here in the US, but also let's say patients here, patients in Europe, patients in Asian countries using multiple data sets.
So, there was a lot of back and forth in terms of not just the ATTRACTION-3 results, but also how we could adequately supplement the clinical trial results with real-world data. But at least in terms of our engagement with FDA, as I said,these real-world analyses were well received as it provided more perspective around the poor prognosis of the disease across regions and how we could make a case around how these trial results could be applicable to US clinical practice. And we were encouraged to have these analyses as part of the final solution package.
Christopher Gayer: Thanks. One more from you Pranav. This one has come in from the audience during your presentation. Did the FDA comment on or was there a consideration on your team's side regarding the differences in racial distribution between the ATTRACTION-3 study population and the Flatiron data?
Pranav Abraham: At least for Flatiron data, there wasn't any. If you look at the racial distribution, the majority of the patients were Caucasians and I think that it gave us an opportunity to show that outcomes are similar respective of racial or geographical locations. And given that we've had a couple of other real-world data analyses where we were able to validate, actually validate some of the findings that we had from the Flatiron real-world analyses. So, as a whole, I think it made a lot of sense to have these multiple analyses, but I just think the real-world analyses as a whole just strengthened and validated results that we saw at Flatiron.
Christopher Gayer: Thanks, Pranav. All right, Lynn, back to you for the hot seat here. I think clearly the poll both at the beginning of the session and toward the end indicates that there's a lot of interest in real-world data as a synthetic control in support of a single arm study. And to your point that you made well during your presentation, the acceptability of real-world data for that particular use has been extremely limited in our experience thus far. And so, I wonder Lynn, from your perspective, what you think we need to see, the agency needs to see, to sort of get to a place where acceptance of synthetic control arms is higher than it is in the current state? What do you think we collectively as a field need to be focused on to sort of move the needle on the acceptability of that use case?
Lynn Howie: I think these first two guidances that have been released this year really speak to the fundamental concern about the use of real-world data and real-world evidence, and that's kind of bolstering and being transparent about what our data quality standards are. And then, also potentially moving to a space where we have some type of standardization of data so that we understand what these data mean across different vendors. And so, I think we still hope that there will be a move to have an adequate understanding of these data as being reliable and high quality enough to be able to serve as a comparator. And we do think too that this will be still in very kind of selective and strategic use cases. Meaning, I don't think, and maybe I'm not overly optimistic, but there's going to be places where there will always be the ability to do a randomized control trial and that will always be the standard.
It's in these spaces where there really is a significant unmet need and significant feasibility issues with performing a randomized controlled trial where I think these use cases will be the places that they can gain traction. But that being said, matching that with data quality so that the regulators can feel confidence in those assessments is going to be critical.
Christopher Gayer: Thanks, Lynn. I think we have about two more minutes before we wrap things up. So, I'd like to do one last question in sort of abbreviated round robin format and I'd like to start with you Kristin, then Pranav, and then hear your take, Lynn. So, the question is what advice would you offer to today's attendees who might be considering using real-world data in a regulatory context? To you first, Kristin.
Kristin Sheffield: So, some of this won't be very exciting, but I'll echo advice that we've heard today and that we've heard from FDA during public meetings. So, it's very important to discuss proposed real-world evidence study plans with them early and follow good procedural practices for observational studies and use those recently released guidance documents to guide our efforts. And I'll also just say that I think that this experience as well as the many recent use cases we've seen have been successful has really decreased some of the uncertainty involved with proposing real-world evidence as part of this submission package. So, I find that it's very encouraging overall.
Christopher Gayer: Thanks Kristin. Pranav?
Pranav Abraham: I think for me, I'm going to repeat what I just mentioned. I think you need to be realistic, be transparent, and I believe, as Lynn mentioned, engage early. I guess that is something that's key, which is prior to submitting the data package to be transparent and show them results along with some of the limitations with real-world data and given that they've had multiple submissions as you laid out, I think regulatory bodies understand real-world data now, the challenge with it. And I think we should be more forthcoming in highlighting those limitations, but also making sure that we can have more meaningful inferences from the analyses that we wish to submit to strengthen our clinical trial results.
Christopher Gayer: Great advice. Anything you'd add, Lynn?
Lynn Howie: I can't think of much to add to this. This is great advice, but I would also just add that remember too, that context matters. And so, contextualizing the reason that you're using real-world data, I think is critical to acceptance.
Christopher Gayer: Thank you all. Okay. One last thing before we wrap up, we want to remind folks in attendance to please stay tuned for our next season of ResearchX - Exploring the principles, promise, and future of real-world evidence coming in March of 2022. And with that, I want to just reiterate our thanks to all of you who've signed in today. Thank you for your time. Thank you to our speakers for their really insightful presentations and advice and insights.
As you'll see in the chat for more content, including past ResearchX sessions, you can visit the Evidence Desk for more information at rwe.flatiron.com. If you have any questions at all about the content that was presented, please don't hesitate to reach out. You can contact us at rwe@flatiron.com. Friendly reminder, please take the survey if you don't mind to help us improve future webinars that should open here soon and just want to invite you all to have a great rest of your day. Stay healthy, stay safe, and thanks for joining us. Take care.