In this episode, hear how life sciences partners have applied RWE to support decision-making across the drug development lifecycle - showcasing its use, value and overall impact.
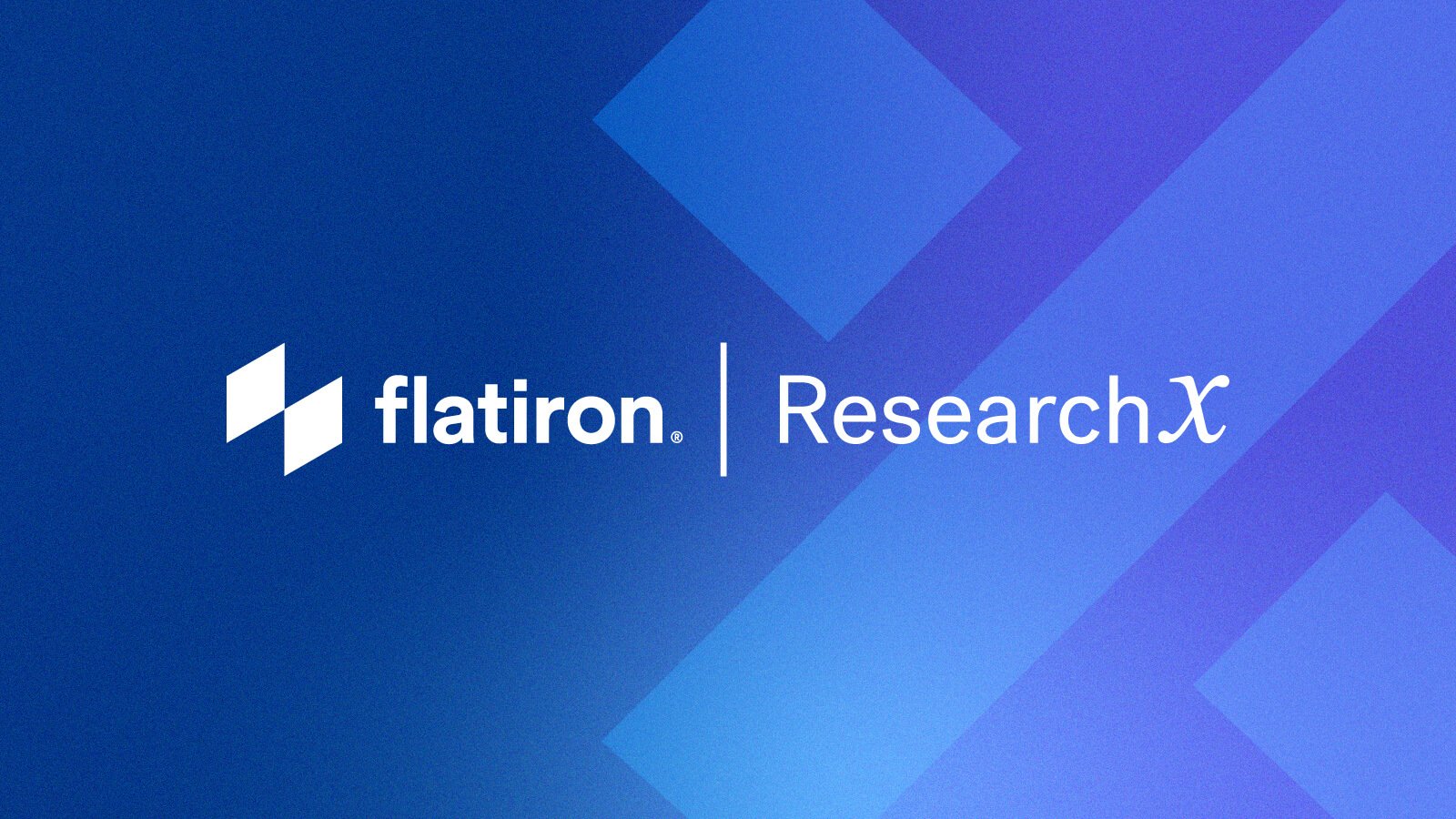
Transcript
Narrator: Previously on ResearchX.
Olivier Humblet: Today we'll extend into analyze showcasing some of the innovative methods that are being used to derive new insights from integrated evidence.
Daniel Backenroth: It's difficult from one source to get enough real-world patients to provide a robust comparator. I'll show how we can use the aggregate method, even when we lack access to all the real-world datasets at the same time.
Katherine Tan: We'll be looking at the application of hybrid control designs using real-world data.
Sanhita Sengupta: We'll be discussing the collaborative effort between BMS and Flatiron Health. The main aim here is to actually reduce the study duration by conducting a hybrid trial.
David Paulucci: Emulation demonstrated that we could potentially reduce the trial by 7 to 11 months.
Jeff Leek: It turns out that machine learning hasn't solved all of our problems, and we have to figure out what we're going to do when the machines don't necessarily give us exactly what we need. We've been really looking at how do you use post-prediction inference, like we discussed from our earlier model, but for real-world evidence.
Olivier Humblet: Very exciting to see that RWD methods are reaching the level of sophistication and rigor we've seen here today. It's really an exciting time to be in this field.
Jyotsna Kasturi: Hi, everyone. Welcome to Episode five of ResearchX! I am really excited to see you all and I am excited to introduce our speakers to you soon. As a reminder, this is part of our ResearchX series, where we've been talking a lot about integrated evidence and how it can really impact the value of real-world data. I'm Jyotsna Kasturi, and I'm in the Quantitative Sciences group here at Flatiron Health and I am excited to moderate the episode today.
For our use cases today, we have our life science partners joining us, and it's really exciting to see how they're using real-world data to make decisions and really impactful uses for improving our patient care.
Let's talk about why we use real-world data. Why are we talking about this? Real-world data as we see can really improve the value of the insights that we generate, help us create and develop new treatments, targeted treatments in rarer diseases or niche population groups, and impact the type of care we can bring to our patients at the end of the day.
So as we think about the product life cycle, real-world data can be incorporated into several aspects and pieces of the product life cycle. For example, from discovery and translational medicine early on to trial designs and clinical development and then regulatory approvals, market access, and beyond. My own experience from pharma has shown me the value of not only using real-world data throughout the lifecycle, but also, seeing the value of integrated evidence starting to play a role.
For example, connecting genomics data to clinical data can be super valuable early on in the discovery or biomarker identification phase, but also help to validate those biomarkers in Phase 3 or later trials and, of course, help targeted therapies be developed.
Now, clinical data and EHR data along with claims data, for instance, can be very valuable to help unlock healthcare resource utilization, thinking about studies where we look at the uptake of a new treatment on the market and really get insight into how patients are using certain treatments. On the other hand, scans data, for instance, can be really valuable and meaningful when incorporated into clinical effectiveness studies, where there may be regulatory facing or HTA studies. We are going to focus on the regulatory and the HTA use cases today. I am excited to hear all of the interesting studies that our speakers have in store for us today.
Let's introduce our speakers. First, we have Fen Ye, Director, Real-World Evidence and Data Science at Novartis. Next we have Hill Hsu, Senior Manager, Center for Observational Research at Amgen. Joining us for Q&A we Victoria Chia, Director, Center for Observational Research at Amgen, and then definitely last but not least, we have Mark Lin, Senior Director, Global Evidence and Outcome Research in Oncology at Takeda.
We have a packed agenda today, but we'll definitely have time at the end for Q&A. Just a quick set of housekeeping reminders for all of you before we get started. We are using the Q&A option within Zoom. At any time, feel free to submit your questions, and we'll make sure to address them. If you have any technical issues or need any help, please contact us through the Q&A option, and we'll do our best to help. Of course, this hardly needs saying anymore. We are all working in a hybrid world, so please excuse any interruptions from our pets or loved ones.
Let's get started. We have a poll for you. We'd really love to hear more about where you would like to use real-world evidence the most across the drug development lifecycle. Would that be early on in the phases where you’re thinking about it in discovery or translational research? Would that be clinical development, regulatory approvals, market access, or in the post-approval setting? Please know that your input will not be visible to the other attendees.
Great. Thanks so much for sharing your feedback and your input here. Let's look at the results. This was really helpful to see how each of you are looking to use real-world evidence as you think of the future in the drug development lifecycle. Let's get started. I'm really excited to introduce Fen, who will speak to us about an interesting HTA use case. Thank you, Fen. Take it away.
Fen Ye: Sure. Thank you. Hi. This is Fen from the Novartis Real-world Evidence Team. Today I'm sharing a case about how RWE could be critical when a drug registration is approved, but the reimbursement was negative. This RWE study was used to support Taf + Mek HTA submission in Canada. Next slide please.
This is a disclaimer of myself and the study. Next slide. First, I will share some background information about BRAF V600E. This is a rare mutation. It only occurs within 1%-2% of patients in the advanced stage of non-small cell lung cancer, specifically in Canada, the new incidence each year is estimated to be about 160 patients.
Regarding this rare mutation, Novartis has a single-arm clinical trial to study the efficacy and the safety for Taf/Mek, either the mono or the combined therapy. Based on the clinical study, FDA approved the combination in 2017. However, in Canada, in 2017, HTA gave negative opinion due to the lack of the comparative data. This provided the opportunity to consider inclusion of RWE comparative data into HTA's strategy. Next slide, please.
For rare mutations or rare populations, it can usually be addressed with a single-arm clinical trial, like Taf + Mek in non-small cell lung cancer. Single-arm trials are generally acceptable for registration purposes, but for reimbursement, it's often considered not enough since HTA and the payer would want to see clinical benefits when compared to available treatment. In Canada in 2017, Taf + Mek submission received a negative HTA opinion with the single-arm trial. And in the 2017 submission, an indirect comparison was included. However, the indirect comparisons from a published trial were not related with BRAF V600E, but there is no published information. In 2019, clinicians and the patient organizations reached out to Novartis and asked us to submit in first-line so that patients can get the treatment option. Then Novartis decided to pursue the submission including RWE comparative effective data. And then we received a positive HTA recommendation in 2021.
Next slide, please. In the comparative data development, Novartis started with an external control analysis, which is to use Flatiron Enhanced Data Mart to select the BRAF mutated patients treated with standard of care from real-world and to compare with the patient treated with Taf + Mek in clinical trials. And with the continued discussion with KOLs and HTA, a real-word versus real-world comparison was requested and added to the comparative packages. So this illustrates the study specifications for both studies. Both studies were published in conference one in April and one in ESMO.
Next slide, please. To adjust for baseline differences, a propensity score model was used. Propensity score weighting was used for both studies so that we can balance the sample and then it’s good for conduct comparison in clinical outcome comparison. Next slide, please. This is an external control analysis. We use the average effect on the treatment to select patients who were similar to those treated within clinical trials with Taf + Mek. This is a patient characteristic before and after the propensity score weighting. More information could be assessed in the ISPOR poster . Next slide, please. This page illustrates a real-world versus real-world comparison, because patients were both treated in the real-world setting, so patients treated with Taf + Mek were compared with those treated with standard care including platinum based chemotherapy, ICI, ICI + PDC. The average treatment effect was used to minimize the potential bias related with treatment selection. Here we presented the post-weighting sample baseline characteristics. The full results were presented at ESMO 2021. And the links were provided for those who are interested in seeing more.
Next slide, please. How did we use real-world evidence and the analysis to support HTA submissions? First, we have the external control analysis. That's the first developed analysis which was the key of the submission. Together with clinical trial information, the real-world analysis provided comparative data for Taf + Mek versus pembro + chemo and Taf + Mek versus chemo result was the main source for the CEA model and our budget impact analysis. So besides our own modeling approach or pure scientific analysis, we also discussed with quite a lot of KOLs, the clinical community, as well as the patient organizations using see the real-world results generated from the ECA analysis. And then as I mentioned, just now, what we discussed with the HTA, there were more queries that came up regarding an additional comparison, like a pembro model compared with Taf + Mek and then they were also interested in understanding how in the same setting the comparative effectiveness will look like.
So we conducted the second analysis in a real-world setting to submit a first-line submission. With the comparative effects in this data from real-world, Taf + Mek received positive HTA recommendation in the first-line non-small cell lung cancer. RWE was used within the package to reduce the uncertainty that comes from the single-arm clinical trial and also helped the HTA make better informed decisions. With this case, I also want to share some of our learning from the Taf + Mek submission process. First, I want to share the real-world data from one geography could be acceptable to support the decision in another. And second, for the real population, external control can be promising to support comparative data and considering the launch time differences globally, real-world comparison could also enrich the comparative evidence packages.
Third, I want to also mention that it is very important to have sophisticated real-world data for the purpose of analysis. And in our specific case, when we started the ECA analysis, we didn't have V600E in the Flatiron EDM. It was then added later. So you can see there are differences in the two study populations. I want to thank all the patients and those who supported our clinical study and I also want to thank Novartis Global and the Canadian team for their collaborative work. Thank you.
Jyotsna Kasturi: Wow. Thank you, Fen. That was really amazing. Congratulations to your team for this really fantastic study. And as you highlighted, I think using real-world data comes with so many exciting features, also challenges and the need to think about methodology. And ultimately using it in an ex-US setting is really valuable to see. Thank you so much. So next we have Hil, who's going to present an interesting regulatory use case where Flatiron's Clinical Genomics Database was used. Over to you, Hil.
Hil Hsu: Great. Thank you for the introduction, Jyotsna. Good morning, afternoon and evening, everyone. Thank you to Flatiron and the organizers for this opportunity to present today on behalf of our Amgen team. I will be sharing our use case of a natural history study, which utilized the Flatiron Health and Foundation Medicine non-small cell lung cancer Clinical Genomic Database, or CGBD, in support of our regulatory filing for Lumakras.
First off, I'd like to provide some background on Lumakras and the oncogenic driver mutation it targets, KRAS G12C. KRAS G12C is found in 13% of patients with non-squamous non-small cell lung cancer. Lumakras, an Amgen drug, is a highly selective oral inhibitor designed to inactivate the KRAS G12C mutant protein without affecting wild-type KRAS. In May of last year, Lumakras was approved by the US FDA, which was less than three years after the first patient was dosed. It has subsequently been approved in three dozen other countries with another 13 global applications currently in review. Lumakras is indicated for the treatment of adult patients with KRAS G12C mutated locally advanced or metastatic non-small cell lung cancer as determined by an approved test and whoever received at least one prior systemic therapy.
As a brief overview of the drug program's history, the planned regulatory approach for Lumakras was to achieve accelerated FDA approval based on a single-arm, phase two clinical trial in this rare disease with high unmet need. During a meeting with the FDA back in 2019, there was a request made for Amgen to provide real-world evidence in the form of a natural history study in order to better understand the disease since little was known about this target patient population. These data would provide context for the single-arm trial data for the Lumakras application without any formal statistical comparisons. Now, at this time, we did not have any in-house real-world data to address this question. So we explored a number of potential new collaborations. We were keeping in mind the recent criticisms the FDA had had for real-world data submitted in support of other accelerated approvals. So our study team had to ensure the data that we acquired was fit-for-purpose, robust and of high quality for the appropriate analysis.
To best describe our population, we needed clinical, molecular and treatment information, as well as real-world outcomes data on progression and death. The molecular data seen in Foundation Medicine's complex panels was key for us to identify patients with a KRAS G12C mutation, as well as explore co-mutations of interest. So paired with Flatiron's comprehensive clinical data, the CGDB benefited us well as a readily available and off-the-shelf data set that met our needs. After thoughtful development of our study protocol and analytic plan, we conducted our natural history study and characterized a cohort of 7,069 advanced NSCLC patients of which 743 had KRAS G12C mutation and were separately characterized.
The study period we assessed was from January 2011 to September 30, 2019. And this audience may already be familiar with this data set, but just to clarify, this data set represents a US population, consisting mostly of community oncology patients. Our study described patient and clinical characteristics, co-mutations, treatment patterns and outcomes, specifically real-world overall survival and progression-free survival. Per our pre-specified statistical analysis plan, we employed the appropriate methods to account for the timing of molecular testing and avoid overestimation of survival due to immortal time bias.
As a brief overview of the study results starting with key patient characteristics, patients with KRAS G12C mutation had a higher proportion of females, never smokers and histologically non-squamous cancers than in the overall advanced NSCLC cohort. The high prevalence of smoking was consistent with the existing understanding of KRAS etiology. Similarly, the finding related to histology aligned with the fact that this mutation has a four times higher prevalence in non-squamous than in squamous tumors. Lastly, the majority of these patients were diagnosed in 2015 and beyond, which is consistent with the availability of molecular testing and surrounding guidelines. With the comprehensive molecular data available, we explored the prevalence of select co-mutations of interest. These insights helped us understand how our drug would fit into the treatment landscape with other actionable mutations, as well as non-actionable co-mutations that had potential prognostic implications. We observed near mutual exclusivity with other actionable mutations, specifically in ALK, EGFR, ROS1 and BRAF. Prevalence of STK11 was higher in KRAS G12C patients than in all advanced NSCLC patients.
Real-world overall survival was assessed in the advanced stage setting from the start of first through fourth lines of therapy, which were readily defined by Flatiron's line of therapy algorithm. We observed similarly poor outcomes with existing therapies in KRAS G12C patients as in the overall advanced NSCLC cohort. Median overall survival was 12 months from the start of first-line and lowered from the start of each subsequent line. With Lumakras indicated for second-line and beyond, these rollout outcomes provided specific estimates on the high unmet need and how these patients might benefit from a targeted therapy. To avoid immortal time bias in these estimates which could overestimate survival, we applied a cohort restriction approach based on the date of molecular testing. Flatiron has helpful guidance on methodological considerations to take when assessing a number of endpoints, which further validated the approach that we took.
In conclusion, there are a few key takeaways from this use case. Originally stemming from a high priority need for real-world data, we were able to foster a new collaboration with Flatiron and utilize their large data source of rich clinical and genomic data representing a substantial proportion of community oncology patients nationally. We conducted a comprehensive natural history study to better understand a rare biomarker defined patient population, advanced NSCLC with KRAS G12C mutation. We developed two publications from this study, which are linked at the bottom of the slide, for those interested. We completed comprehensive study reports, which were included in the new drug application for Lumakras, which was filed in December of 2020. In the resulting FDA's multidisciplinary review document following approval, Amgen's real-world evidence was included and the FDA stated their agreement with Amgen's description of the existing standard of care, specifically the second-line treatment options for advanced NSCLC patients.
Fast-forward to today, nearly a year following the FDA approval, we continue to utilize Flatiron data for the program, monitoring the treatment landscape as it evolves and addressing a broad range of scientific questions of interest. Thank you all for your attention. And this concludes my presentation.
Jyotsna Kasturi: Thank you so much, Hill. That was amazing. Your presentation was a great example of using multiple sources of data and in a targeted therapy situation. Next we have Mark, who will present on an interesting regulatory submission study, using Flatiron data with quite an innovative trial design. And just a quick reminder to everyone, please continue to submit your questions and we'll get to Q&A soon. Thank you so much.
Mark Lin: All right. Thank you. Good morning, good afternoon, everyone. This is Mark Lin from Takeda. It's my pleasure to present a case study, on how we used real world data, such as Flatiron data at Takeda, to help with regulatory and HTA submission for the most recent approved drug, Exkivity or the generic name mobocertinib, for non-small cell lung cancer with EGFR Exon 20 insertion mutation.
Just sharing some background for the audience . A couple of the previous speakers also talked about non-small cell lung cancer, and as most of you know, non-small cell lung cancer is a heterogeneous disease that can be divided into multiple subtypes depending on the biomarker expression.
These biomarkers determine different treatment options and outcomes. EGFR Exon 20 counts for a small portion of non-small cell lung cancer. At the time when we initiated the study, there was no drug approved specifically for this subtype and the patients with this mutation responded very poorly to the previous generation of EGFR TKI, that targeted a more common EGFR mutation.
Takeda developed mobocertinib, which differentiates from other commercially available EGFR TKI by specifically binding to the kinase domain of the EGFR Exon 20 insertion mutation. The clinical trial has shown a rather promising efficacy of the drug. It's a single arm trial. We have a response rate ranging from 28% to 35%, depending on the IRC or investigator assessment and PFS of 7.3 months or OS of 24 months. And I think really it just comes down to, is this data meaningful? As I mentioned, this is a single arm trial. The nature of single arm trials, combined with a rare population, the lack of standards of care and historical control, present challenges for both regulatory approval and also market access approval, as well. So we decided to utilize real-world data to show an unmet need and to also to provide comparative evidence of the drug, to support drug approval and market access. So, after discussing with internal and external stakeholders, we selected both a US and ex-US database for the real word analysis. For today's talk, I will focus on the US database called Flatiron Spotlight. It was chosen because it's fit for purpose. You have the right population, good sample size, and also the unstructured data that really covers the primary endpoint of the trial, which is the overall response rate. Two main types of analysis were conducted. One is called a benchmark analysis, which is more like a natural history study, that does not include any statistical adjustment of the patient's baseline characteristics. Here we show that the OR is about 14%.
And we also look at the benchmark analysis of the PFS, and overall survival, which is 3.3 months, and 11 months respectively. There was no standard of care, as I mentioned, and this outcome is numerically worse than the trial, showing the unmet need of the current treatment. And another analysis we ran, we call indirect comparison. But it's really just comparing the clinical outcome between the trial and real-world data, after a statistical adjustment of the baseline characteristics. The different agencies could have different preferences on the waiting method, or the benchmark analysis. So, we applied a waiting method at the IPTW, to match the prognosis factor. Such as age, gender, smoking status, time to diagnosis, and so on.
And we then look at the clinical outcome, before and after the waiting. As you can visually see here, for the kaplan-meier curve, the analysis shows that mobocertinib had a better response rate, a better progression-free PFS and overall survival than the real world control, with or without weighting. So, these results really confirm the unmet need of the current treatment and support that mobocertinib has substantially better efficacy than the current treatment. So, to appreciate the real-world effort, I want to share with you a timeline of the regulatory engagement. We actually started to have the conversation with the FDA back in 2018. Almost three years before the NDA submission. And later, we submitted the protocol to the FDA for review. And some of the preliminary data we generated was also used for breakthrough designation, mainly 0.2, the appropriate comparator for the benchmark purpose.
We really have engaged with the FDA multiple times. And the analysis was part of the NDA submission. And for other regulatory and HTA submissions as well. So finally, last September, we got approval from the FDA. And we also just recently got approval of mobocertinib by the UK this March. Last month. It's good news for the patient who has this diseaseThis is the only oral target therapy for this particular rare disease, with great unmet need. We're still waiting for the position for other regulatory agencies, and HTA bodies as well.
Some of the key learnings are that the success of using the real world data approach is really a team effort. You need support from multiple teams, including clinical, regulatory, the global outcome group and the statistical function, etc.
Second is that you need to proactively engage with regulatory agencies. I mean, the earlier the better, in our case. Of course, there are definitely some lessons learned for both sides. I think the guidelines, or the concept of how we use real-world data, was also evolving from the regulatory agency side as well. But we have to respond quickly, right? Every time when there's an additional request, we really have to respond in a very short period of time.
And the third is that we need to choose a database that aligns with the population. Fit for purpose, with good quality. And we have to do tailored analysis for different agencies. And, last but not least, as I mentioned, some agencies could require a slightly different analysis. So, this is also something to keep in mind. It's not like one size fits all. I think this is my last slide. I'm also going to thank the patients and colleagues at Takeda for supporting this work. And also of course, thanks to Flatiron, for providing the data. Thank you.
Jyotsna Kasturi: Thank you, Mark. That was amazing. It was really interesting to see how much planning goes into using real-world data in a study and how we think about the methodology, and all of the data considerations to bring us to a stage of decision making. So really, really exciting and congratulations to the team.
So, as we think about all that we've heard today, I hope that it really starts to make sense and it's more concrete for all of the attendees today. We've talked a lot throughout the ResearchX episodes about integrated evidence, and how to use real world data for various use cases and hopefully today with our great speakers, you've been able to see some actual decision-making, and how real-world data was incorporated into their whole drug development life cycle. So, if we think about tying all of this together, there are a couple things that come to mind for me.
One is really the value of real-world data that's been demonstrated through some of these use cases. In not only highlighting and bringing new insights to the table, but also really impacting oncology care for our patients by bringing the right treatment to the right patient at the right time with targeted treatments. A few particular points that came through throughout all of the presentations today for me was, one, how real world data can supplement and augment our clinical trials and really help us learn from more patients. But also help us see a more general group of patients for global decision making, as we've heard today.
Second, again, how real world evidence, when thought about as integrated and incorporated into the drug life cycle, can really accelerate our decision making with regulatory authorities or HTAs, and therefore help bring access to patients much faster.
And lastly, again, bringing it all back together is the concept of integrated evidence. And how we can use multiple sources of data that are often complementary to each other, to really enhance the data and make it more robust. So, I've been in this field for a while, and it's exciting to see the progress that's being made, and the promises and the power of real-world data being demonstrated. Thank you again to all of the speakers. Last reminder, please submit your questions and let's jump into Q&A. Okay, great. So we are seeing quite a few questions that have already been submitted. The first one's for Fen. The question is, how did the team think about the generalizability or transportability of patients, when using US real world data for ex-US or Canadian use? For example, when the team thought about cohort selection or the competitor treatments that were available in different geographical markets? I would love to hear your thoughts on that.
Fen Ye: That's a great question. I would say this should be evaluated case by case and it also depends on the market. In Canadian HTA submission the accessibility on foreign data is higher than, maybe, in other regions. So it definitely depends on the region. Second, because this is a real mutation, it plays a critical role, because the data within the locale is also hard to get. There are also similarities between the US and Canada in patient characteristics, as well as treatment practices. And lastly, we are assessing clinical outcomes. So that's what we also use, the appropriate method to weight, such as propensity score, weighting to minimize the bias. So, within all this discussion with the HTA, as well as the local commission, this is an acceptable case. And we encourage the discussion with the local regulation, or the agencies to increase the success rate.
Jyotsna Kasturi: Thank you Fen, that's really helpful. The next question is for Hill. This is an interesting one. A common challenge in using real world evidence for rare cohorts, is the limited sample sizes available. How did the Amgen team account for low counts in their planning process? Ranging from initial feasibility testing, to positioning of the analysis, in their regulatory submission?
Hil Hsu: Thank you for the question. I think this disease is rare, but given the overall size of the CGDB advanced NSCLC cohort, we were able to pull out quite a decent sample size of patients. So, at the time of the publication, this was actually the largest cohort of KRAS G12C , advanced NSCLC patients. So, when paired with the level of information that we had on the data set, we were able to paint a fairly reliable picture of this cohort. But I think this question about limited sample size is particularly relevant for another natural history study that we actually completed with Flatiron's CGDB data. Which was in the metastatic CRC space, colorectal cancer. Which has a much lower prevalence of KRAS G12C . And so, we had a really small sample to work with. And in order to ensure that we had retained as many patients as possible in our sample for our outcomes analysis, we used a different approach than what I presented today.
Instead of a cohort restriction, we used a delayed entry model. So, this allowed us to address and avoid immortal time bias, but allowed us to keep as many patients in as possible, to preserve our sample size. And this specific work was recently accepted as a manuscript. So it should be available in public domains soon.
Jyotsna Kasturi: That's amazing, I'm excited to read it. And there's so much novel methodology that we need to think about when using real world data. Thank you. We have a question for both Mark and Vicky. Welcome back, Vicky to our Q&A session. There was a lot of interesting discussion about different trial designs through both of the case studies that were presented. When choosing an external or historical control, what do you think is the most challenging, since you'd want to use it for regulatory agencies?
Mark Lin: I always say, everything is challenging! You really need to find the right sample size. Then, I think one of the other speakers spoke about this, whether it's US-based, or it's non-US-based, you'll definitely hear different comments from different agencies. Some clearly say, "We would like to see some non-US-based data." And then, the second thing is missing data. I'll start with the outcome. For our trial, the primary endpoint is response rate. I think this is not something that is typically captured by a standard database. They probably have PFS and OS, but those PFS, OS, they could be informative, but it's not really the same as the response rate.
So then, you have to find a data source that does capture those endpoints that are relevant for the trial. And also, you probably need some literature or some other things to validate the real-world data that's similar to what we have seen in the trial setting. Because for the OR, they have reached this 1.1, a different way of measuring. I think we'll take a little bit extra effort to explain how those endpoints are relevant. And the third thing is that, you still want to match the baseline as close as possible. You can only match what you have.
For example, we tried to match the things that are available. We have age, gender, and brain metastasis, and so on and so forth, but there's certain things you just cannot match. For example, in a US database, the vast majority of patients are white. But if your trial is some kind of lung cancer trial that is very largely Asian, you're going to have a disproportionate percentage of Asian patients. This is not something you can really match. You can only conduct some sensitivity analysis, some subpopulation saying that. Maybe a different population would not change the conclusion. I think it's a lot of things, a laundry list. But I think it really comes down to, have to plan this a little bit early, have the buy-in from the company, from different groups, and have the conversation with the regulatory agency frequently and early.
Victoria Chia: Yeah, I would agree with a lot of the points that Mark stated. Sotorasib is our second experiment with using real-world data to support regulatory drug applications. First was Blincyto. I think a lot of our experience on using these types of studies to support regulatory applications have been really ensuring, like what Mark said, that data is fit for purpose. And so, are you looking at the right population? Are you covering the geographic regions that you potentially need to cover? Do you have rigorous exposure and validated outcomes data, as well as stability, like Mark said, to make sure you can adjust for important co-variants. I think all of these things are really necessary in order to conduct these studies well. As well as what Mark said, having early talks about all of these data sources, how you're going to use them within your company, as well as with your regulatory agencies, FDA, EMA. Are you doing natural history study versus true comparative analyses? I think all of those things are really important to plan ahead, to make sure studies are successful.
Jyotsna Kasturi: Thank you for sharing. A second part of that question for both of you, and probably all of you is, can you speak to the logistics in the process when you were dealing with the FDA, and how do you think through some of that as you bring a project to a regulatory agency?
Victoria Chia: I guess just to start from Amgen's side, I think we had meetings with them fairly early in the drug development process, and often, our protocols, SAPs were sent to them ahead of time before studies were conducted. I think definitely, those sorts of interactions. And you can see with the new guidelines, the regulatory agencies like FDA, they want to see that.
Jyotsna Kasturi: Early engagement is important.
Victoria Chia: Yeah!
Jyotsna Kasturi: Thank you. Anything you'd like to add, Mark?
Mark Lin: Yeah. I think we just try to leverage any opportunity when we have a conversation with the FDA. Because during the application process, you have different meetings and they are probably driven by clinical. They're talking about the different outcomes and different ways of thinking. We always try to add real-world data-related questions. It's part of the same package. It's not that we're going to have separate meetings just talking about real-world data, but somehow leverage the opportunity, since the teams are going to have a meeting with the FDA anyway. We try to incorporate some of the questions. Because really, there are multiple opportunities to discuss with the FDA. We have the breakthrough designation meeting, then we have a pre-NDA meeting. You can always try to incorporate some of the questions.
Jyotsna Kasturi: Thank you so much. That's really helpful. We have the next question for Fen. Different HTA bodies may have different preferences or expectations for the use of real-world evidence in their global dossiers. What did the Novartis team do to tailor their submission to the CADTH, and increase the likelihood for success?
Fen Ye: We worked together with our Canadian team with their global approach, tailored to local access. Many discussions are engaged with HTA in Canada, to meet the requirement from the HTA, but also we educate the HTA definitely to consider the specific situation, as well as the strength of the information. We are more open in discussion with the HTA regarding what is our proposal and what are the limitations, however with this realm of observation, there is no fixed history clinical trial. Real-world data did provide valuable information as another aspect to consider.
Jyotsna Kasturi: Thank you, that's helpful. I know we're running out of time, so we have one last question for the panel. What is the greatest learning that you have had from working with real-world evidence on your study? It's a big question.
Fen Ye: I want to cite a phrase saying, "It takes a village to raise a child." It also applies to the RWE science development. It is not only the life science company, not only the real-world data providers, but also the community designator who will benefit from it, who will be using this, including the clinical experts, the patient organizations, the HTA, HA. All who are included, can make this a process more robust, and also being understandable to the audience who we are facing.
Jyotsna Kasturi: Thank you, Fan. Hil we'd love to hear from you.
Hil Hsu: Yeah, definitely. If I had to sum up the greatest learning from this study, I would say, just taking a step back and realizing the huge value there is in real-world clinical data linked with the genomic data set, just ready, off the shelf. You don't need to wait for any additional abstraction. This linkage was really key to let us develop and conduct our study fairly quickly and smoothly.
Jyotsna Kasturi: Thank you. Mark?
Mark Lin: I think a lot has been said already. The whole real-world data thing is definitely still evolving. That's why you keep seeing the new guidelines from different regions. FDA, EMA, and China have their own guidelines. I think you just need to be agile, and really respond to whatever the new requirement is quickly. I won't be surprised if the current guideline changes in the next few years again. Maybe different qualities, or maybe a different standard. But I think now, it's a good starting point. People start to realize the value of real-world data. I think we just need to keep learning, keep adapting to new things.
Jyotsna Kasturi: Exactly right. Thank you. Vicky.
Victoria Chia: Yeah, I think communication is really key, like Fen said, not only within your company, with the regulatory agencies, as well as all the healthcare providers, patients that you're serving. But I think we talked about fit for purpose data, and making sure your data is suitable to the question you're trying to answer. And then finally, making sure you have rigorous analytic methods. Those are all great learnings and takeaways from our experiences.
Jyotsna Kasturi: Yeah, I really appreciate all of the feedback. Thank you all so much for sharing your learnings and your really interesting case studies with us today. I think it really shows the impact of real-world evidence. I'm excited for the future of real-world data.
Victoria Chia: Thank you.
Mark Lin: Thank you.
Jyotsna Kasturi: As we move forward, this wraps up Episode Five, and I am really excited for the last episode for our ResearchX season, which is Episode Six, and that's on May 11th. Please put a reminder on your calendars. The episode will focus on a very important aspect of the whole real-world evidence journey, which is the patient perspective. That will be a panel discussion with our US Patient Advisory Board, and our UK Patient Voices Group. Last but not least, we probably couldn't get to many of your questions, so please continue to reach out to us. Please contact us at rwe@flatiron.com if you have more questions or you'd like more details on the content presented today. And again, I want to thank all of our speakers, thank you so much, and thank you to all of the attendees for joining in making this a fun event. Stay safe and healthy.