Given the recent release of various guidances from global health authorities, the regulatory use of real-world evidence (RWE) continues to evolve. At the same time, using RWE to support regulatory submissions continues to be a key focus area for both regulators and life sciences companies. In this ResearchX session, industry leaders shared their experiences with regulatory applications of RWE in various contexts and discussed how RWE can meaningfully contribute to regulatory submissions. Specific case studies were also shared.
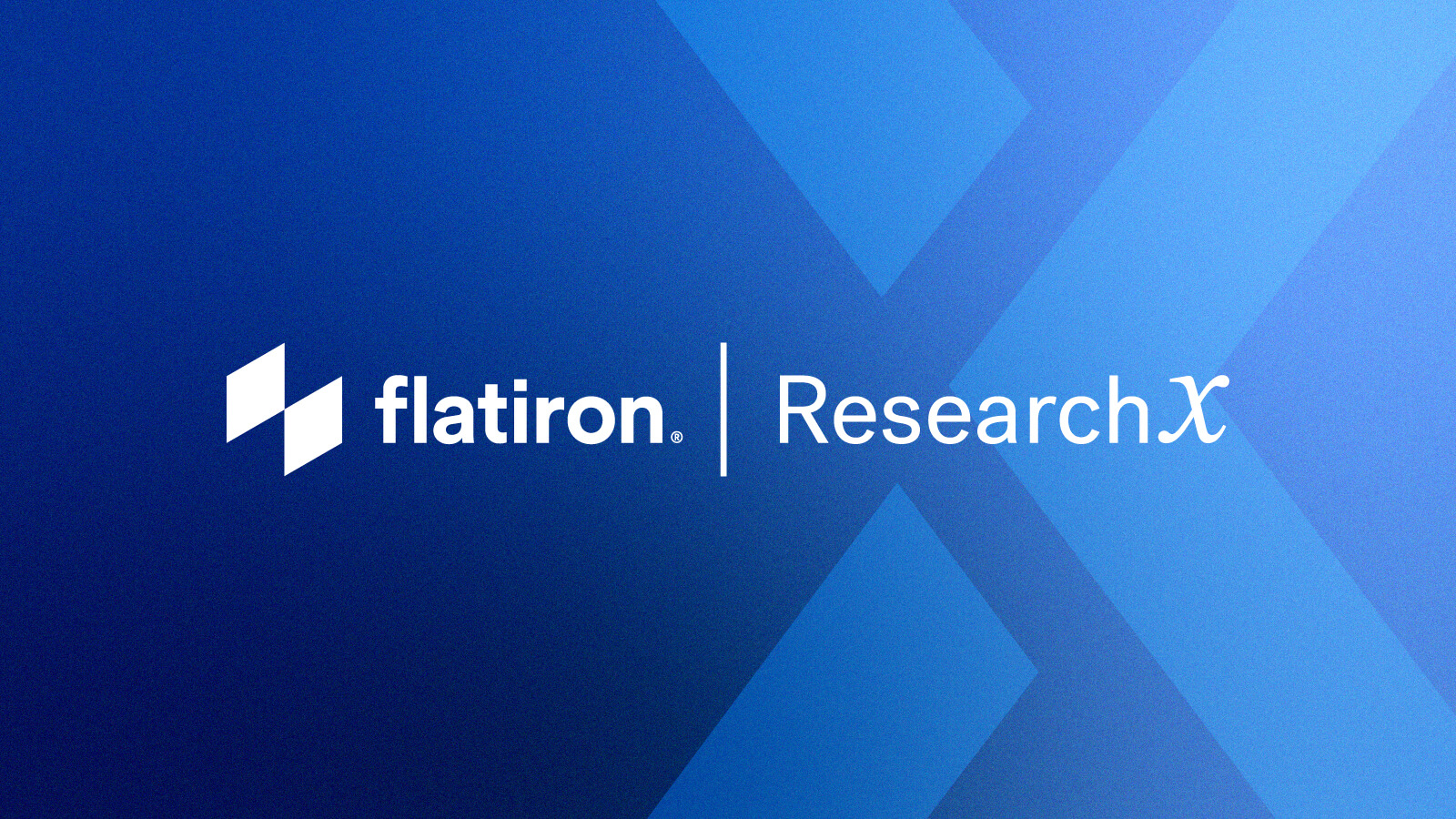
Transcript
Jillian Rockland:
Hello everyone, and welcome to ResearchX. We're so excited you've joined us today. We're looking forward to an interesting hour where we're going to dive deep into the evolving landscape of fit for purpose, regulatory, real-world data and evidence, sharing perspectives and concrete experiences. My name is Jillian Rockland and I'm a Director on Flatiron Health's Real-World Evidence team. It's so wonderful to see so many of you from across the industry in attendance.
I'm excited to introduce our speakers who will help bring this session to life. First, I'll share some background on recent RWE related guidances from health authorities and some key considerations. Next, Brian Clancy, Director, Real-World Data Solutions at Foundation Medicine will discuss using clinical genomic data to support a postmarketing commitment. Evgeny Degtyarev, Director of Biostatistics at Novartis will discuss leveraging RWE to contextualize a single arm trial. And lastly, we'll have a panel discussion with all the speakers and bring in Lynn Howie, Medical Director II from Flatiron to join the conversation about the regulatory landscape and our evolving perspectives.
And while we have a packed agenda, we'll still have time at the end of the session for audience questions. Before we get started, a few quick housekeeping items. I'd like to draw your attention to the Q&A option available throughout the webinar. Feel free to submit a question anytime, or to reach out to us afterwards if you'd like to discuss any of today's content in more detail. If you have any technical questions or issues, please let us know via the Q&A tool and we'll do our best to help. And finally, please excuse any interruptions from pets or loved ones. Given the hybrid working world, many of us are working from home.
Before we get started, we'd like to learn a little bit more about you. You should see a poll pop up on your screen momentarily. Our poll question is, which of these applications of RWD has your organization historically considered or incorporated into regulatory submissions? Select all that apply. The choices are: Characterizing natural history or unmet medical need, As an external comparator to a single arm trial, Satisfying post-marketing commitments or requirements, To expand a label into new indications, For global market access including HTA, or None of the above. A couple more seconds, and let's close the poll and share the results. Thanks everyone for providing your input. It's really helpful to get a pulse check on how your organizations have historically looked at using real-world data for regulatory purposes. And I'm excited for you to learn more in the case studies that you'll hear today.
And now I'll dive into today's session. As many of you know, over the past five years real world evidence has been a focus of FDA and global health authorities. In the US, following the mandate outlined in the 21st Century Cures Act, FDA released a draft framework for RWE in 2018 that contained information on the use of RWE and regulatory submissions, including scenarios in which RWE may provide evidence in support of a regulatory decision. Recently in 2021, FDA released a number of draft guidances on the use of RWE that touch on important considerations for submission and data standards, fit-for-purpose use of data and diversity plans.
Outside of the US, we're also seeing regulators provide guidance or perspectives around RWE regulatory applications. The HMA EMA joint Big Data Task Force released a summary report in early 2019, which reviewed the landscape of big data and identified opportunities for improvement. In January 2020, the task force released its phase two report, which proposed 10 priority actions to enhance its ability to collect, manage and interpret data, and this includes building sustainable capacity and capability for real-world data and advanced analytics. In 2021, EMA explained their plans to establish methods and data standards for high quality collection and use of RWE. Most recently, in 2022, EMA commenced the creation of the data analytics and real world interrogation network, or Darwin EU, an EU-wide network allowing access and analysis of healthcare data from across the EU to drive the conduct of RWE studies.
As I mentioned, over the past year and a half, we've seen the release of several FDA draft guidances that speak to the use of RWE, highlighting FDA's commitment to clarity and transparency around their expectations. These guidances focus on recommendations related to the use of specific data sources, including EHR, medical claims and registry data, data standards, general considerations for the use of RWE for marketing applications and potential use of RWE to support diversity plans. Many of these guidances have core commonalities, most notably FDA's clear and consistent focus on ensuring that data is fit-for-purpose regardless of use case, highlighting the importance of pre-specification of study protocols and analysis plans, and finally, that transparency around the real-world data used to support safety or efficacy claims is a critical prerequisite to success. In thinking alongside the guidances, we think it's important to consider regulatory use cases for RWD on a spectrum. On one end, there are more traditional uses of RWE as evidence to inform regulatory strategy. An example would be leveraging RWE to provide justification for a comparator cohort when designing a clinical trial. On the other end of the spectrum are more aspirational use cases where we see the potential to leverage RWE as substantial evidence to inform a regulatory decision.
But this requires addressing key data and methodological limitations in particular when considering retrospective data. A recent example of a successful substantial evidence use case was the FDA's approval of tacrolimus to prevent organ rejection in adult and pediatric patients receiving lung transplants. This approval, which used prospectively collected registry data, reflected how a well-designed non-interventional study relying on fit-for-purpose, real-world data can be considered adequate and well-controlled under FDA regulations.
In the middle of this spectrum, we see a breadth of opportunities for real-world data to be used as supportive evidence contributing to the totality of evidence to form a regulatory decision. While fit-for-use, RWD is dependent on both the clinical and regulatory context. There's significant near term potential for supportive RWD for regulatory use. A little later today you'll hear two case study examples of just that.
Before diving into those case studies. I'll talk a bit more about core principles of successfully leveraging RWD for regulatory applications that we've gained from our deep experience working with sponsors and FDA and that we see reflected in recent draft guidances. Start with why, engage early and often and be transparent. Starting with why. Without clearly and coherently justifying why RWD is the right fit for your application, your use case could really fall flat in early conversations. Unfortunately, the argument alone that RWE can be generated more quickly and less expensively than an RCT simply isn't going to cut it. Based on regulatory discussions and feedback to date. There are certain circumstances in which RWE is best suited to support trial data. When cohorts of interests are small, making randomized trials and feasible where unmet need is significant and available approved therapies are limited, ill-defined or highly toxic or invasive, when there is an expected large effect size from preliminary data, or where a body of evidence from other data sources, including controlled trials already exists in a related population or tumor type, providing a convincing argument to health authorities as to why RWD is an important part of your application that can't be filled by other means, is key to success.
Next, engaging early and often. There are clear recommendations throughout several of the FDA guidance documents that highlight the importance of adequate planning, feasibility and pre-specification. Sponsors should work to proactively decide the best strategy for RWE use based on development planning. Collaboratively developing the RWE regulatory strategy, protocol and statistical analysis plan with your data provider can allow for the most robust planning and discussion with health authorities. Submitting and/or discussing your plans with health authorities ensures that time and resources aren't expended on RWE studies that won't add overall value to your application and allows you to work with your data provider to adjust, interpret and respond to regulator feedback to ensure that there's confidence from any conclusions that are drawn from your RWE study. Ideally, this process would be iterative, collaborative, and occur throughout planning.
Finally, last but definitely not least and a point and I really want to drive home today: be transparent. Real-world data, like any data source, has its strengths and limitations. Being clear on those and what conclusions can be reasonably drawn from the data are very important. Ensure that if needed or requested, the patient level data is available. Clearly describe all data processing and translation approaches used to generate the data set. What this means is a clear, concise and accurate description of how the data is curated from the source data, all the way to the final analytic dataset. Openly discussing potential limitations as well as strategies for mitigation. In every study, regardless if it includes real-world data, there's a potential for confounding and bias. Clearly articulate these situations and proactively propose a plan for mitigation that's specific to real-world data and includes the most up to date methodologies.
Finally, be clear about data quality considerations, meaning that health authorities have faith that the data is fit-for-purpose. There's a common misconception that there's a binary yes/no data quality bar for real-world data for regulatory use, and frankly, that's just not the case. All data can be used for varying purposes. Evaluating whether the data is fit-for-use to address your specific regulatory question involves assessing whether key data elements and a sufficient representative sample size are available. And considering the accuracy, provenance, traceability and completeness of the data, it's important to articulate to regulators your considerations for why this data is a fit for your particular use case.
Overall, we're continuously learning and adapting to new guidances and frameworks issued by health authorities, along with learnings that we gain from examples of successful and unsuccessful use of RWE. Data that is fit-for-purpose, both relevant to the research question and can answer the question reliably is key to regulatory success, and the bar for RWE can be higher the less supportive and more substantial the evidence becomes. Robust and timely planning to use real-world data is extremely important, and leveraging the expertise of your data provider can be helpful in designing and framing the real world study and responding to health authority feedback.
Lastly, transparency is critical. In order to make a determination on the fit-for-purpose nature of the RWE and conclusions drawn from it, regulators must be clear about the source of the data, data processing and transformations and the analyses. And now I'm going to turn it over to my panelists. Today, you'll hear about the application of these guidance related considerations for two supportive evidence use cases. One is part of a post-marketing commitment and the other is supportive to a controlled trial. And now Brian Clancy, Director of Real World Data Solutions at Foundation Medicine will discuss leveraging clinico-genomic data for companion diagnostic post-approval studies.
Brian Clancy:
Thank you so much Jillian! I am so happy to be here and I am also so excited to be talking about what we're going to be talking about today, which is really, how might access to high quality clinico-genomic data enhance the probability of regulatory success for our companion diagnostic.
Foundation Medicine was founded on the reality that cancer is a disease of the genome, and if we could generate a greater understanding of the cancer genome, then we can generate insights that will help patients today but also help patients tomorrow. The hallmark manner that Foundation Medicine delivers our insights are in a series of diagnostic reports that list a series of treatment options that may be applicable to a particular patient, based on their genomics.
One opportunity for identifying a treatment option is really in the context of a companion diagnostic. Now, these companion diagnostics have an important role in the life cycle of a new drug, where health authorities, particularly the FDA often require that a new drug targeting a biomarker specific population, has an FDA approved diagnostic called a companion diagnostic in order to gain or maintain approval by the FDA or other health authorities around the world.
Today we'll be specifically talking about a companion diagnostic for the drug entrectinib. So in June 2022, the US FDA approved the Foundation One CDx assay to be used as a companion diagnostic for two indications for brand name, Rozlytrek, or entrectinib. The first of these indications was for NTRK gene fusions across all solid tumors. The second, which will be talking about in greater detail today, is for ROS1 fusions in non-small cell lung cancer.
Now, ROS1 gene fusions are quite rare, generally occurring in only 1% to 2% of NSCLC diagnoses. Given the rarity of ROS1 in NSCLC, there is of course limited clinical research data on ROS1 mutated NSCLC. To this end, while clinical trial samples were evaluated to show ROS1 CDx patients may benefit from entrectinib therapy, there is interest in how to enhance that evidence base, even beyond those clinical trials. In this context, a series of discussions with the FDA led to discussions around how the Flatiron Health-Foundation Medicine Clinico- Genomic Database or CGDB may be able to support some of those incremental real-world evidence generation needs. The result including the successful approval of the ROS1 CDx was, but as a condition of that approval, Foundation Medicine intends to conduct a post-approval study powered by the Flatiron Health-Foundation Medicine CGDB. So we look forward to generating real world evidence of the effectiveness of the companion diagnostic to identify those ROS1 patients who may benefit from entrectinib therapy.
So why CGCB? A few different reasons here. First, the CGDB offers attributes consistent with relevant regulatory guidance such as the traceability of that real-world data. The second is a track record of successful regulatory use cases of the CGDB, including in the context of natural history of disease studies. A third, and particularly important for this particular use case in the world of companion diagnostics is our ability to revisit sequence data that was generated in the past with our updated FDA-approved companion diagnostic biomarker definition and associated analytical software. We believe that this feat of applying today's diagnostics to retrospective samples is critical for providing the fit-for-purpose data for this particular use case, given how important it is to define that biomarker positive cohort precisely, one might even say perfectly. So this feels to us like an important step forward in balancing a series of public health goals.
Obviously, we want, as a society, new therapies, new diagnostics to be available for patients. But in some of these rare diseases such as ROS1 NSCLC, there's always going to be an interest in enhancing the amount of evidence and particularly potentially the real-world evidence that these diagnostics do indeed identify those patients who may benefit from therapy. The availability of real-world data is another option for answering those questions. For generating that type of evidence really bridges those public health goals that we all have. A few perspectives coming out of this case study. The first is that as we endeavor to really fulfill the promise of precision oncology, we are going to inevitably create rare patient populations, in this case, ROS1 patient populations. Whenever we create these rare patient populations, the likelihood that tissue availability or the number of patients in a clinical trial could potentially create a challenge, is going to be higher. And in this context, the option to be able to augment clinical trial data with real-world data and the ability to generate real-l world evidence is going to become and continue to be an important option for a number of stakeholders in this world. The ROS1 CDx approval is one example, whereby having that incremental optionality of being able to deploy the real-world data to be able to create that real-world evidence does have the potential to improve the probability of regulatory successthat in this case, a companion diagnostic does indeed get approved by the FDA.
Just a little bit about CGDB. This is essentially the overlap of Foundation Medicine’s genomics data, and the Flatiron Health electronic medical record data. With that, I will turn it over to Jillian.
Jillian Rockland:
Thanks so much, Brian for sharing that really interesting post-marketing use case. A quick reminder to the audience to remember to submit questions via the Q&A tool at the bottom of the screen that we'll answer later. And now I'm going to turn it over to Evgeny, who will talk about leveraging RWE to contextualize a single arm trial.
Evgeny Degtyarev:
Thank you Jillian! Hello everyone, and apologies for my voice and possible cough, as I got a cold a few days ago. So I will share today with you an example where we used real-world evidence to contextualize a single arm trial in oncology. Randomized trials remain the gold standard for providing evidence for regulatory submissions, but single arm trials have been used in settings where randomized trials are infeasible or unethical to conduct, in particular in rare diseases, often in settings with high unmet need and last line of therapy. And also if the investigational therapist were providing early signs of response in the Phase 1 study. And so as you see here, the numbers for approvals based on single arm trials in oncology are quite substantial and are applicable to both EMA and FDA. And ELARA was such a single arm trial which was a study enrolling patients with follicular lymphoma after at least two prior lines of therapy.
The investigational therapy was tisagenlecleucel, which is an anti-CD19 CAR-T therapy approved in three hematologic indications. I will not go into details of the therapy because it's not relevant for my talk, but what is important to know is that CAR-T therapies are personalized cell therapies requiring manufacturing after enrollment. So what it means is that you can see on the slide is that during the screening procedure there is this material is collected and it is used after the enrollment of the patient into a clinical trial to actually produce the CAR-T product, which can take multiple weeks. And then, the patient is infused once the product is available.
And when we submitted the protocol for this trial to our EMA Rep to the Norwegian Health Authority, we actually received a very clear request to provide external control data. As you can see here, the Norwegian Health Authority data that “we assume that prior to any comparative analysis, the external control will be pre specified and consist of a population where there is access to individual patient level data”. And they also highlighted that the selection criteria for this external control should match the selection criteria for the patient population in the ELARA trial.
We already anticipated the need for patient-level data and an external control, especially from the payer perspective. And so we already planned from the beginning a retrospective chart review study called ReCORD follicular lymphoma that was conducted mainly in Europe but also in some US sites and only in the academic centers, and most of these centers also participated in our clinical trial. And in addition to that, later we decided to conduct an electronic health record study with Flatiron, which is a US-only database and includes only community centers. So we felt this two sources, one community centers in US, the other one mainly Europe and mainly only academic centers, would actually provide a nice complimentary package of real-world data, and it would allow the reviewers a robust assessment of efficacy of tisagenlecleucel in follicular lymphoma patients.
As it was requested by the health authority, we went to the CHMP Scientific Advice before conducting the study and before knowing the results of the real-world evidence, and we asked them several questions. Of course, first whether it would be adequate to actually file the single arm trial, considering the results from the indirect comparisons with these two sources. But then also, we went into the details of the two real-world data sources, and so we asked them whether the CHMP Scientific Advice considers it adequate how the cohort are selected in both sources, whether the quality of the two sources and how the data is collected is actually adequate to support regulatory submission and whether the real-world endpoints are suitable in our case.
And here, of course there are some differences because in lymphoma clinical trials, we use the so-called Lugano criteria, which is quite complex criteria which includes an assessment based on PET-CT, but also bone marrow assessment and in real-world data, because not everything is available and so there are some differences to be expected in this case. We also asked them questions about our methodology and our approach to define the question of interest using target trial and estimated frameworks, and also our planned analysis to address this question of interest and I will come back to this point a bit later. So here you see the feedback of the CHMP Scientific Advice, and they confirm to us that indeed, in this particular setting, follicular lymphoma is a rare disease with a very fragmented therapeutic landscape. It would indeed be useful for them to understand the efficacy of tisagenlecleucel if we can provide this contextualization of the single arm trial with indirect comparisons. They also highlighted that, while real-world evidence is supportive, they do require compelling results from pivotal trial for successful approval. I think this is fair feedback and something that we also expected.
They agreed with our external sources, highlighting again that the credibility of the comparisons will depend on the sample size, the completeness of the data, the comparability of the study populations, the ability to adjust to important prognostic factors, and also the endpoint definitions. And again, I would like to emphasize here that at that time we actually didn't know the final sample size. We didn't know whether certain variables would be missing or not. We really went for the scientific advice very early. We had, of course, some visibility at that stage and we knew roughly what to expect, but still, there were some surprises when we got the data as well.
They had some useful comments with regard to sensitivity analysis and also they asked us to restrict the data collection period for real-world evidence accounting for the last relevant EMA approval in particular, and also they currently use the efficacy criteria, the Lugano criteria that I mentioned previously. And they also endorsed the use of target trial framework and proposed methodology. And I wanted to quickly discuss this framework because I think it is very helpful actually to reach some clarity and interactions, first internally with all the functions involved in such effort, and it is a truly cross-functional collaboration including clinical real-world evidence experts, in market access experts, the regulatory experts within the company, statisticians of course as well. But also clarity with external stakeholders such as regulatory and payers. And it also helps to understand all the limitations in there. I fully agree with Jillian's point previously that transparency is key and we really need to be open on the limitations.
And so the target trial framework, basically forces you to think about target randomized clinical trial that you would've liked to conduct in an ideal world where it would be possible. And so that's the left side of the table where you then describe this ideal target randomized trial based on some key elements such as the population, the treatment, the endpoints, and so on. And then on the right side, you describe what is possible to be emulated using your single arm trial and the real-world evidence cohort. And that allows you at quick glance to see the differences between the randomized trial that you would've conducted and your indirect comparison, and also to structure the discussion in the meetings, but also then in the writing in your submission documents, for example. Because then in the subsequent sections, you can comment for example directly on the differences for eligibility and explain which inclusion-exclusion criteria in the real-world evidence cohort were feasible to implement, which were not feasible to implement and what could be the potential impact of this. And similarly for other factors as well, it's very easy to see the differences and also a good way to structure the discussion.
And it also helps you to avoid potential biases. For example, I mentioned before that there are two important time points. One is the CAR-T therapy, one is the enrollment date, which basically from a single arm trial perspective, this would correspond to the randomization date in the randomized trial. And then in the real-world evidence cohort, there is no prescription date, which would be the ideal responding time point for the randomization date. But based on the therapies that are used in this setting, we could assume that the standard of care treatment start is actually very close to the assignment. And clearly in this case, to use the infusion date of the CAR-T therapy would actually bias the results and would not be proper emulation of a randomized trial, and so we used the enrollment date here.
So in the end, tisagenlecleucel was approved in this indication. We actually thought that because it was considered useful by our apperture , that this information, the real-world evidence in direct comparisons, would also be useful for the prescribers, so we suggested to share this data also in the EU label, but this was not accepted by the EMA. However, in the EPAR, in the Public Assessment Report, there's quite a lot of information about the assessment of these two indirect comparisons and this is just a summary statement here where they agreed that it provides valuable context and is deemed supportive for the pivotal trial, despite some uncertainties. And in particular, with these uncertainties, they were referring to inability to emulate some of the inclusion criteria in the ELARA study, some prognostic values missing and also the differences in the response criteria as I mentioned before. And we currently have the submissions to the payers ongoing using the same approach and the same sources, and some additional sensitivity analysis were also performed to account for some missingness in the prognostic factors as well.
So to conclude, definitely in this case, I think we have seen that real-world evidence was relevant for regulators and payers. I think there are multiple areas where we need more guidance from regulators, but I think in particular also more clarity about the role of real-world evidence for drug labels would be welcomed. It clearly takes a cross-functional collaboration within the companies, and I think in our case, most of us had worked for the first time with real-world data, and certainly it was a significant time commitment and it's something important to consider as well. And this is why early planning and regulatory consultations are also critical. There are tools that can help you to actually facilitate transparent and structured discussions about real-world data sources, about their limitations and pros and cons in general, and it can help you to avoid biases and I think we should be using this target trial and estimate frameworks more often in future. And certainly further dialogue between sponsors, regulators, payers, but also academia I think is needed to develop best practices and guidances in particular for sensitivity analysis, but also for other important errors. And I think I just wanted to share a few references. The first three are more on the clinical side. They're describing also the results. And the last three are more about the methodology, in case you are interested to read more about the target trial framework or the estimate frameworks that we used. And with that, I'll hand it over to Jillian.
Jillian Rockland:
Thanks so much, Evgeny for that presentation. So before we move on to our moderated panel, we'd love to take one last poll. This last poll is more future-focused. So over the next one to two years, which regulatory applications of real-world data do you think your organization will pursue? Select all that apply: Characterizing natural history or unmet medical need, As an external comparator to a single arm trial, Satisfying post-marketing commitments or requirements, To expand a label into new indications, For global market access, including HTA, or None of the above. I'll give the audience 10 more seconds.Okay, let's end the poll and share the results. It's really interesting to see how you all envision the future use of RWE for regulatory applications. Thanks so much for taking the time to share. So now we can move into our panel discussion. So I will start with a question for everyone. We can start with Evgeny, then Brian, then Lynn. FDA, EMA and others have released various global guidances. How has your thinking evolved since the emergence of these new guidances?
Evgeny Degtyarev:
Sure, I can start. I think, I mean, in general, we have to acknowledge that the use of real-world evidence for decision making by regulators is certainly an evolving field, and health authority approvals based on real-world evidence so far are, and mostly I think to expand an indication in already established therapies . I think in my company, we had a recent example with Alpelisib in PIK3CA-related overgrowth syndrome where the approval was granted fully based on real-world data. But I think such situations are still rare, and mostly we are using it to contextualize single arm trials. And so from that perspective, the health authority guidances in particular, the FDA guidances at the end of last year were certainly helpful to set the expectations and definitely contributed to better understanding of regulatory requirements.
I think at the same time, it didn't particularly change my mind. I think real-world evidence was always a high bar. It remains a high bar and I think the FDA guidance has clearly reinforced this message and just provided more clarity on various aspects. But at the same time, I think there are still open questions. I know many companies, many industry working groups actually submitted questions and comments on the guidances asking for clarification of different aspects, and so we are waiting for those clarifications. As a statistician, I'm also eagerly waiting for the design and analysis guideline by the FDA that has not yet been released as well, and that will be important to extend their thinking. So I think overall, it's a good start. It's definitely important to have this guidance. Some things became more clear, but certainly we need more guidances in future as well and more dialogue in general, more opportunities for dialogue.
Jillian Rockland:
And now we'll hear from Brian.
Brian Clancy:
Thank you so much. And Evgeny, thank you so much for your response. Yeah, in reading the guidance, I think that the primary thing that I took away and our organization took away is reconfirmation of the importance of having traceable real-world data. And there are a number of unique aspects to genomics real-world data that, when we're thinking about how we want to manifest that and the big investments we're making and continuing to manifest that, the guidance gives us a lot of confidence that the investments that we're making are the right ones to be making in this particular field. So happy to pass to the next person. I think Lynn is next, right?
Lynn Howie:
Yes, thank you. So I think the guidances, in addition to echoing what Brian said about being very clear about how we curate and come up with our data and being clear in communicating that to regulators also helps us to be very laser focus on three key components, and that's better understanding how we characterize the prognostic features of our patient populations, how we characterize the exposure to therapy that our patient populations received, and then how we characterize and understand outcomes in a setting that is heterogeneous and not kind of well controlled like a clinical trial. So I think that the guidances has really helped us to kind of laser focus into the areas that are going to be of key scrutiny when understanding real-world data in a regulatory setting.
Jillian Rockland:
Wonderful. Thank you so much all for sharing your perspectives there. So for the next question, this one for Brian and Evgeny specifically, how did you think about designing your study to ensure that the real-world data was fit-for-purpose? I know we heard a lot of folks mention that kind of as a buzzword. Can we start with Brian?
Brian Clancy:
Sure, happy to. Yeah, unfortunately there's not too much I can say at this time about the design of our post-approval study, but what I can say is that we think of fit -or-purpose from the perspective of the data and the perspective of the analysis. First, the data needs to be reliable and relevant. We need to have our genomics real-world data trace back to our sequence data and our medical device, and we also need to be able to identify the patients in our retrospective databases who are ROS1 CDx positive in
the same exact manner of that FDA approved device. In terms of the analysis, it needs to be a pre-specified analysis. It needs to address the evidence generation gap or need that is being laid out by our interactions with the relevant health authorities. In this case, generating more evidence that ROS1 patients do indeed have the potential to benefit from entrectinib.
Jillian Rockland:
And Evgeny?
Evgeny Degtyarev:
Yes. I can add from my perspective. Well, first of all, we need to be very clear on the question of interest and actually the purpose. And this may take some time. It's not so straightforward sometimes really to define precisely what we want to answer with our real-world data source and then to find actually the proper real-world data source to address our question of interest. And as I mentioned, I found this framework quite useful where you really just think about your question of interest, then in the second step, think about the randomized trial that would have addressed your question of interest in the ideal world, and then in the last step then you compare this ideal, randomized trial with the indirect comparisons that you're planning to perform with a single arm trial and the external control arm from real-world data and you really critically appraise whether this real-world data source is fit-for-purpose and whether the available data is relevant and sufficient.
And so really kind of facilitating a transparent discussion, being open about potential sources of bias and the limitations of these sources and then trying to understand the process, concept of different sources.
Jillian Rockland:
Wonderful. Thank you. So I'll move into our last couple questions. The first starting off with Brian and then Evgeny. We talked a lot about the opportunities for real-world data to support regulatory applications. What do you see as both the biggest challenges and opportunities for regulatory RWE?
Brian Clancy:
Yeah. Thanks for the question. The biggest challenge with real-world data, it's never going to be the same as clinical trial data, but that's also the biggest opportunity for real-world data. I won't enumerate all the benefits of real-world data to this expert audience, but there are real strengths there, not just weaknesses, to these real-world data sets. There's strengths in those differences. So having clinical trial data, as well as real-world data is only going to diversify your options for creating great evidence, answering more questions in different ways and answering questions that health authorities are interested or increasingly interested in the answers to. So I think that's the big opportunity, is really embracing not just the weaknesses of real-world data but really the strengths relative to other options.
Evgeny Degtyarev:
Yeah. I think from my perspective with regard to the challenges, it would be useful to have some consistent, harmonized real-world evidence framework by all regulators and really a bit more alignment in the regulatory thinking there. It's certainly because companies are doing the development plans globally, and certainly it is not useful if something is welcomed by maybe FDA but not by the EMA and vice versa. And certainly more alignment there would be helpful. And we do see sometimes differences in how regulators perceive real-world data as well. And the same applies also for the prescribing information where a bit more clarity is needed on when real-world evidence would be considered relevant for the prescribers and why do we see real-world data represented everywhere at clinical congresses but it's not part of the labels. I would think that if I see it everywhere at clinical congresses, it is relevant for the physicians, but somehow regulators don't see it this way. And it is not clear why at least. I think these aspects need to be discussed, but certainly it will take time I think realistically. It is difficult to harmonize this process and really to clarify all the open questions at this stage. The opportunities, I see I think multiple errors where certainly real-world evidence can play a major role in drug development. I think we do see more and more challenges in conducting randomized trials, particularly in some rare disease settings with approved gene therapies or cell therapies, where it is quite difficult to conduct a study, for example, of the next generation CAR-T therapy versus the first generation CAR-T, which is already standard of care, because you expect incremental benefits, because in such head-to-head study, you may face a risk of bias due to some manufacturing challenges in the comparator arm and some other programs.
And so there are some alternatives that have been actually recently presented in some statistical forums by some colleagues where you actually randomize to a single arm trial or to a real-world data. And so you're using real-world data and keep the randomization. And so there are some innovative approaches certainly that I think we need to explore more on the methodology side. I think definitely the post-approval commitment is a big topic. I think Brian's presentation nicely shows it, but it's certainly something that can be explored more in particular settings where we have surrogate endpoints and maybe an opportunity to get an early approval based on surrogate endpoints than to have post-marketing commitments that require more time and more patience but can be done in the real-world setting. And so that's another, I think, opportunity.
And then the third one would be the hybrid designs where, for example, we have two to one randomization and we complement the control arm with some real-world data. I think we have seen recently some of the examples also in regulatory setting but certainly I think these designs could be used more in future as well and could also help to have more efficient development plans. And so yeah, I think there are quite a lot of opportunities I would say, but all of them require close partnership between industry and regulators in future and more dialogue I think.
Jillian Rockland:
Awesome. Thank you so much. Also, we've gotten lots of great questions from the audience. So I'm going to move on to asking some of those. The first one's for all panelists. And maybe we can start with Lynn. So this is a real easy one. "What will it take for the industry to cross the high bar set from FDA and EMA on acceptability or use of real-world data beyond contextualizing data in the confirmatory trial setting?"
Lynn Howie:
So that is probably the $10 million question. And I think relating back to where we feel like those rare patient populations with significant unmet need and, like Evgeny also said, where there's clear evidence that doing a standard randomized controlled trial would be difficult, if not impossible, I think are the likely first places that that might occur. That being said, we still have to recognize that substantial evidence is substantial evidence and where a randomized controlled trial can be done, real-world data is not likely to replace that. And so I think we have continued deep aspirations but also understand the evidentiary needs of regulators in the process and want to ensure that we're providing regulators the best data available to them to help make their decisions.
Jillian Rockland:
Thanks, Lynn. Maybe Brian, anything to add?
Brian Clancy:
Not too much. Agree with everything that Lynn said. Having real-world data is going to provide options, but it's not going to be a silver bullet option in the very near-term. But there are so many questions that take place outside of kind of the core objectives of a particular filing that can be engaged on when you have that real-world data, when you have the option to use it. And you can't eat an elephant all at once. You need to kind of chop it up into pieces, is what I hear. I've never eaten an elephant. But I think that's really what our industry is trying to do right now, is, "Okay. Where are the pieces that we can bite off with real-world data? What are those questions that we can answer?"
Jillian Rockland:
Evgeny, anything to add?
Evgeny Degtyarev:
Not much to add. I guess I would just say that, I mean, we all need to learn and the best way to learn is to have case studies, and so someone has to start. And even if it takes some time just to generate some case studies within each company to get experience to give also the regulators a chance to have some experience with real-world data and then, yeah, to progress on this topic.
Jillian Rockland:
Awesome. So moving on to the next question, this one's specifically for Lynn. "What is Flatiron Health working on to address the concerns raised in the guidances?"
Lynn Howie:
So as soon as those guidances came out, we started to really work to make sure that we were addressing everything as clearly and accurately as we could in the near-term. So as Jillian talked about, being clear about where our data come from and how those data are curated, generated is critical. We have worked to provide our clients a number of regulatory documents that can be submitted along with their submission to help explain, give the data story for Flatiron data when it's used in a regulatory use case. We're working to expand our network to include those patient populations, as Evgeny spoke about, academic populations and broader populations outside our primarily community based network to better generate those cohorts of rare patient populations, as well as trial-like cohorts.
We're also working to partner our data with other data sources such as claims data to be able to better help deal with issues of missing data, as well as to triangulate and give confidence in those co-variates exposures and outcomes, and just working to continue to engage regulators both with our clients, as well as through research collaborations in an effort for us to better understand regulatory needs, as well as for regulators to better understand where our data come from and to give them the confidence that they need to be able to use our data for regulatory decision making.
Jillian Rockland:
Thanks so much, Lynn. So we have time for one more quick question. The next one is for Evgeny. So, "Did Novartis consider pooling trial data with RWD and then conducting analyses using statistical adjustments?
Evgeny Degtyarev:
So if the question is whether we really performed an indirect comparison assessing, for example, the difference in PFS between our single arm trial and the real-world evidence source, then yes, we were adjusting also for difference in baseline characteristics, for example. So this was done. We have never considered to pool these two different sources with each other due to various differences between them and we performed the two analysis separately, one versus Flatiron and one versus ReCORD.
Jillian Rockland:
So it looks like we're really close to time. So since we weren't able to get to all of your questions, please know that the lines of communication are always open even after we end the episode. And feel free to get in touch with us at rwe@flatiron.com. Friendly reminder to please take the survey upon closing. It'll help us improve future webinars. And also our next ResearchX, “Scaling Insights In Cancer Care with Machine Learning”, will take place on Thursday, December 1st. Stay safe and healthy. And thank you so much for joining.